作者:哈工大SCIR 钟蔚弘
1. 简介
随着预训练模型的发展,研究者也开始尝试将预训练模型的架构和方法应用于多模态任务当中。在图片-文本多模态任务当中,预训练模型的应用已经取得了出色的表现。相比于图片,视频内容中包含的信息更加丰富而冗余,多帧之间可能包含高度相似的画面。与图片不同,视频内容中自然地包含了时序信息,随着视频时间长度的增长,其包含的时序信息也愈加丰富。同时,由于视频数据的体积相较于图片而言也更加庞大,数据集、模型的构建都为研究者提出了更大的挑战。因此,如何更优雅,高质量地建立视频-文本表示之间的联系、进行良好的交互,并为下游任务带来提升,就成为了研究者们探究的问题。
本文简单梳理了当前视频-文本预训练的模型架构及相关数据集,同时,针对视频信息较为冗余的特点,对引入细粒度信息的工作进行了简要介绍。
2. 常用预训练数据集
多模态预训练的数据通常来源于大规模的模态间对齐样本对。由于时序维度的存在,视频当中包含了比图片更加丰富而冗余的信息。因此,收集大规模的视频-文本对齐数据对用于视频预训练存在较高的难度。目前,大部分研究者所使用的公开预训练数据集主要包括HowTo100M[1]和WebVid[2]数据集,此外,由于视频和图片特征的相似性,也有非常多工作利用图片-文本预训练数据集进行训练,本节主要对视频-文本预训练中常用的数据集进行简单的介绍。
2.1 HowTo100M
学习视频-文本的跨模态表示通常需要人工标注描述的的视频片段(clip),而标注一个这样的大规模数据集非常昂贵。Miech[1]等人发布了HowTo100M数据集,帮助模型从带有自动转写的旁白文本(automatically transcribed narrations)的视频数据中学习到跨模态的表示。HowTo100M从1.22M个带有旁白的教学(instructional)网络视频中裁切得到了136M个视频片段(clip)。视频的教学内容多由人类展示,包含了超过两万三千个不同的视觉任务。
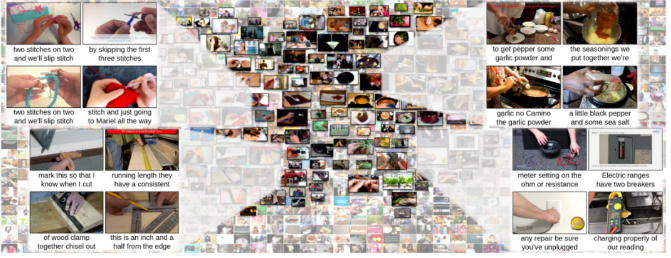
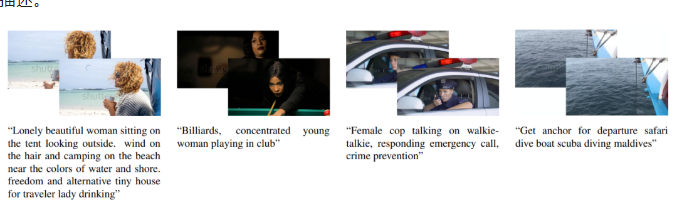
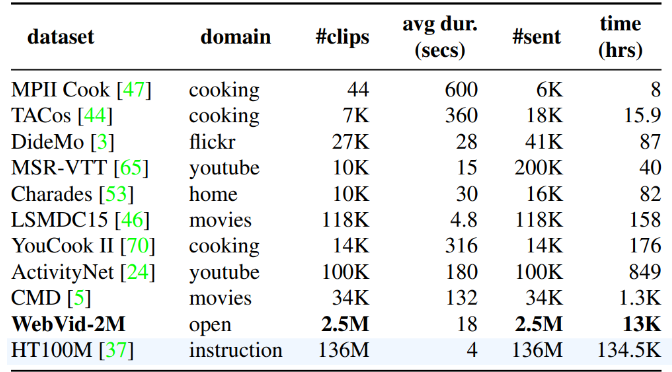
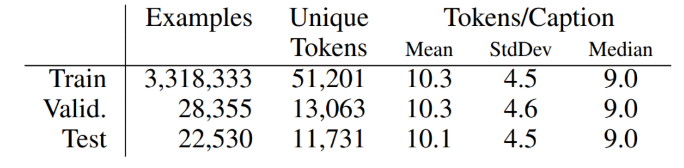
表3 Conceptual Captions的统计数据[3]
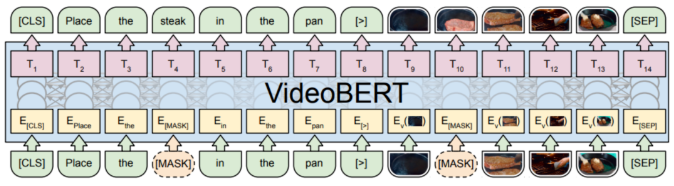
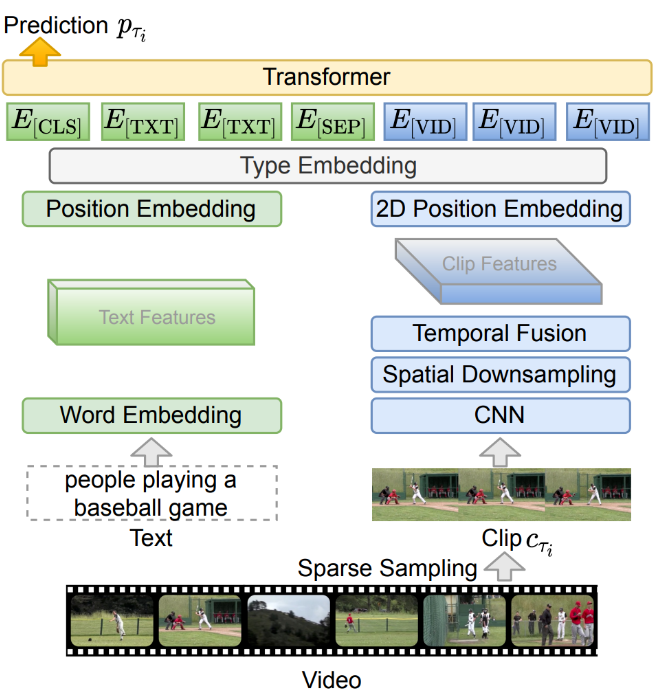
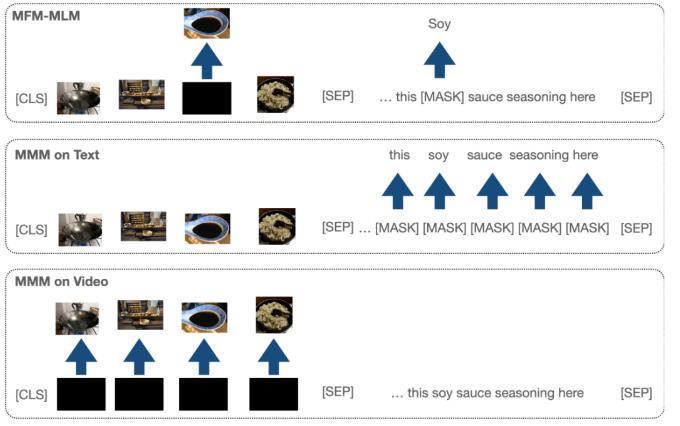
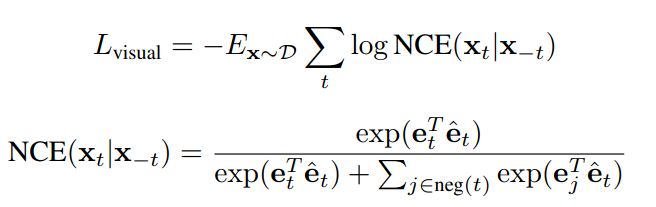

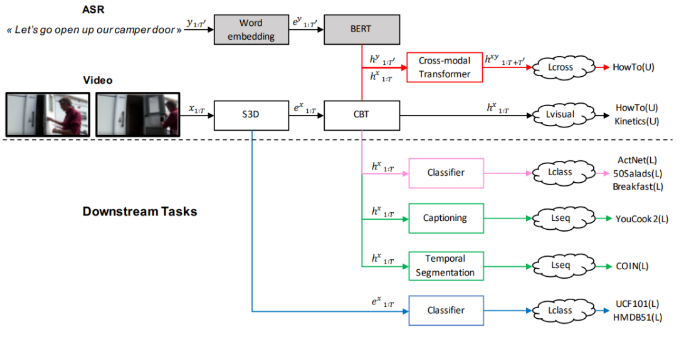
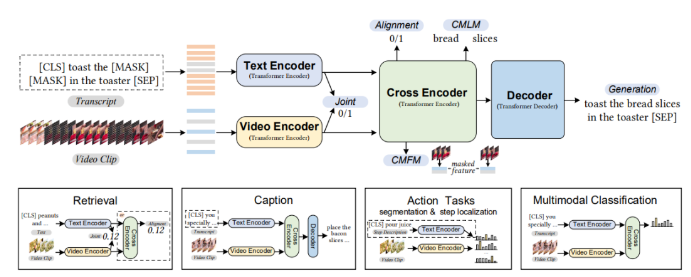
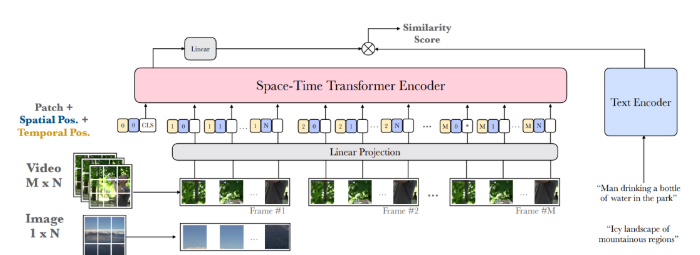
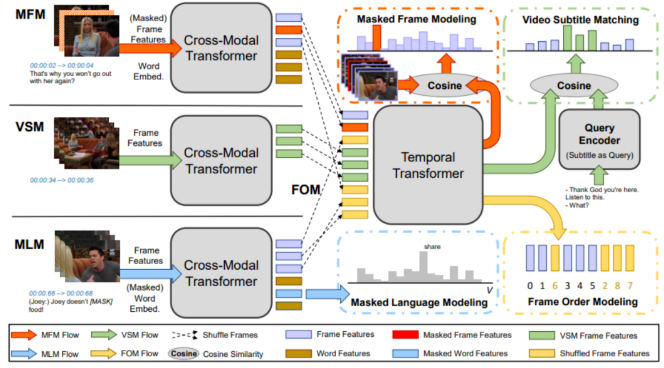
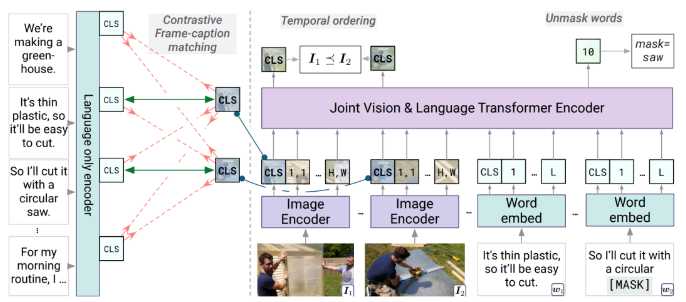
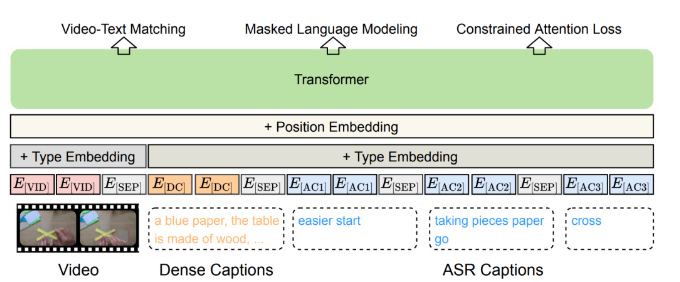
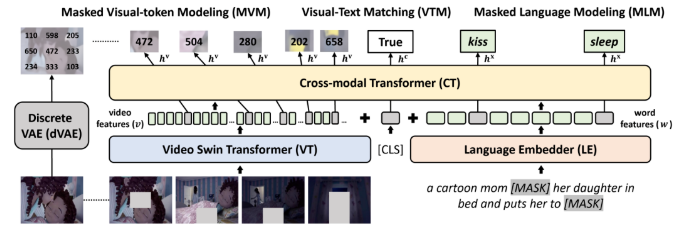
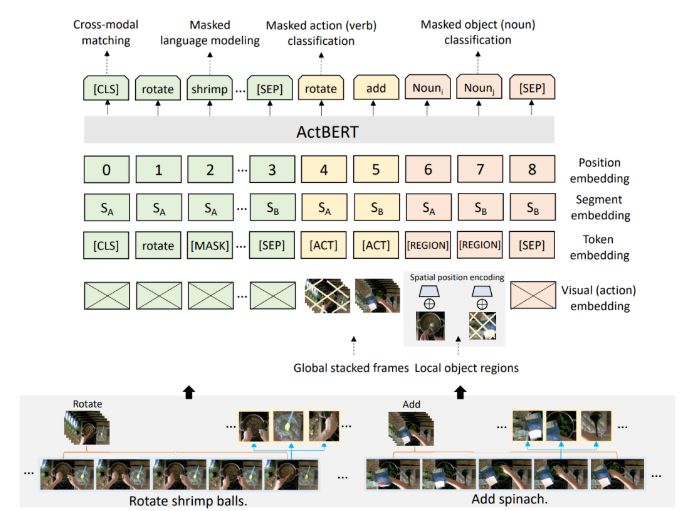
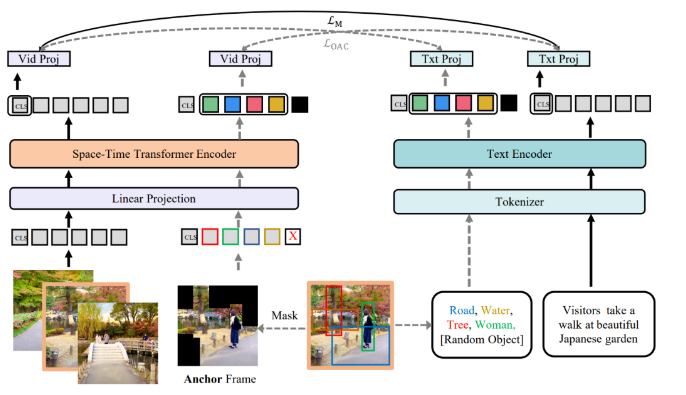
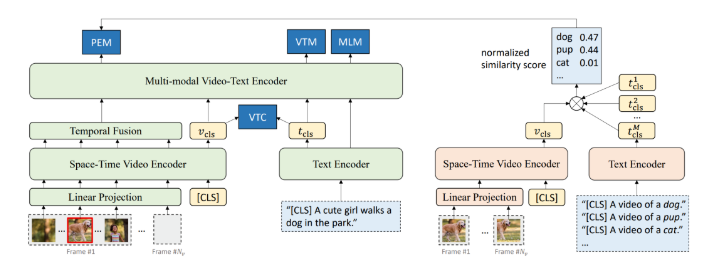
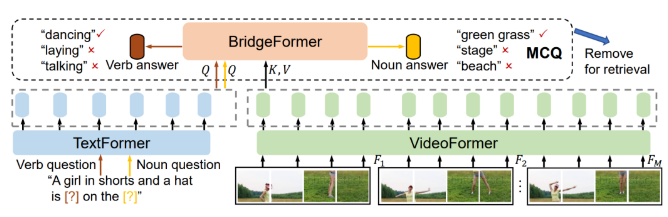
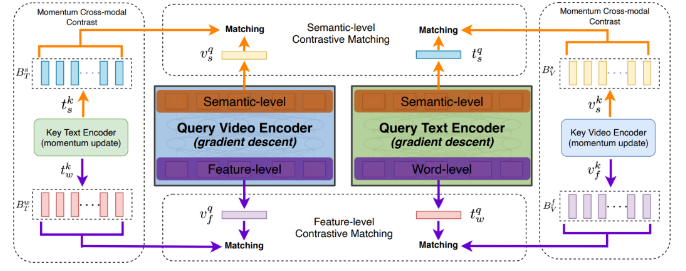
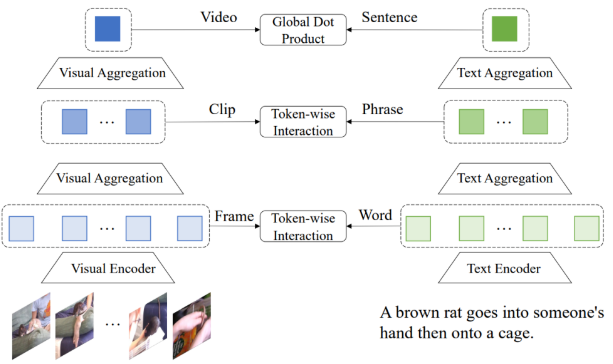
[1] Miech A, Zhukov D, Alayrac J B, et al. Howto100m: Learning a text-video embedding by watching hundred million narrated video clips[C]//Proceedings of the IEEE/CVF International Conference on Computer Vision. 2019: 2630-2640.
[2] Bain M, Nagrani A, Varol G, et al. Frozen in time: A joint video and image encoder for end-to-end retrieval[C]//Proceedings of the IEEE/CVF International Conference on Computer Vision. 2021: 1728-1738.
[3] Sharma P, Ding N, Goodman S, et al. Conceptual captions: A cleaned, hypernymed, image alt-text dataset for automatic image captioning[C]//Proceedings of the 56th Annual Meeting of the Association for Computational Linguistics (Volume 1: Long Papers). 2018: 2556-2565.
[4] Sun C, Myers A, Vondrick C, et al. Videobert: A joint model for video and language representation learning[C]//Proceedings of the IEEE/CVF International Conference on Computer Vision. 2019: 7464-7473.
[5] Devlin J, Chang M W, Lee K, et al. Bert: Pre-training of deep bidirectional transformers for language understanding[J]. arXiv preprint arXiv:1810.04805, 2018.
[6] Lei J, Li L, Zhou L, et al. Less is more: Clipbert for video-and-language learning via sparse sampling[C]//Proceedings of the IEEE/CVF Conference on Computer Vision and Pattern Recognition. 2021: 7331-7341.
[7] Xu H, Ghosh G, Huang P Y, et al. VLM: Task-agnostic video-language model pre-training for video understanding[J]. arXiv preprint arXiv:2105.09996, 2021.
[8] Sun C, Baradel F, Murphy K, et al. Learning video representations using contrastive bidirectional transformer[J]. arXiv preprint arXiv:1906.05743, 2019.
[9] Luo H, Ji L, Shi B, et al. Univl: A unified video and language pre-training model for multimodal understanding and generation[J]. arXiv preprint arXiv:2002.06353, 2020.
[10] Bertasius G, Wang H, Torresani L. Is space-time attention all you need for video understanding?[C]//ICML. 2021, 2(3): 4.
[11] Li L, Chen Y C, Cheng Y, et al. Hero: Hierarchical encoder for video+ language omni-representation pre-training[J]. arXiv preprint arXiv:2005.00200, 2020.
[12] Zellers R, Lu X, Hessel J, et al. Merlot: Multimodal neural script knowledge models[J]. Advances in Neural Information Processing Systems, 2021, 34: 23634-23651.
[13] Tang Z, Lei J, Bansal M. Decembert: Learning from noisy instructional videos via dense captions and entropy minimization[C]//Proceedings of the 2021 Conference of the North American Chapter of the Association for Computational Linguistics: Human Language Technologies. 2021: 2415-2426.
[14] Fu T J, Li L, Gan Z, et al. VIOLET: End-to-end video-language transformers with masked visual-token modeling[J]. arXiv preprint arXiv:2111.12681, 2021.
[15] Zhu L, Yang Y. Actbert: Learning global-local video-text representations[C]//Proceedings of the IEEE/CVF conference on computer vision and pattern recognition. 2020: 8746-8755.
[16] Wang J, Ge Y, Cai G, et al. Object-aware Video-language Pre-training for Retrieval[C]//Proceedings of the IEEE/CVF Conference on Computer Vision and Pattern Recognition. 2022: 3313-3322.
[17] Li D, Li J, Li H, et al. Align and Prompt: Video-and-Language Pre-training with Entity Prompts[C]//Proceedings of the IEEE/CVF Conference on Computer Vision and Pattern Recognition. 2022: 4953-4963.
[18] Radford A, Kim J W, Hallacy C, et al. Learning transferable visual models from natural language supervision[C]//International Conference on Machine Learning. PMLR, 2021: 8748-8763.
[19] Ge Y, Ge Y, Liu X, et al. Bridging Video-Text Retrieval With Multiple Choice Questions[C]//Proceedings of the IEEE/CVF Conference on Computer Vision and Pattern Recognition. 2022: 16167-16176.
[20] Liu S, Fan H, Qian S, et al. Hit: Hierarchical transformer with momentum contrast for video-text retrieval[C]//Proceedings of the IEEE/CVF International Conference on Computer Vision. 2021: 11915-11925.
[21] Min S, Kong W, Tu R C, et al. HunYuan_tvr for Text-Video Retrivial[J]. arXiv preprint arXiv:2204.03382, 2022.
[22] Van Den Oord A, Vinyals O. Neural discrete representation learning[J]. Advances in neural information processing systems, 2017, 30.
内容中包含的图片若涉及版权问题,请及时与我们联系删除
评论
沙发等你来抢