要了解未来会是什么样子,研究我们的历史通常会有所帮助。这就是我将在本文中要做的事情。我回顾了计算机和人工智能的简史,看看我们对未来有什么期待。
我们是怎么到这里来的?
世界变化有多快,即使是相当新的计算机技术对我们今天来说也感觉很古老,这一点变得清晰起来。90年代的手机是带有微小绿色显示屏的大砖头。二十年前,计算机的主要存储是打孔卡。
在短时间内,计算机发展如此之快,并成为我们日常生活中不可或缺的一部分,以至于很容易忘记这项技术是多么新近。正如时间轴所示,第一台数字计算机大约八十年前才被发明出来。

我提到的第一个系统是忒修斯。它由克劳德·香农(Claude Shannon)于1950年建造,是一种遥控鼠标,能够找到走出迷宫的路,并可以记住它的路线。1七十年来,人工智能的能力取得了长足的进步。
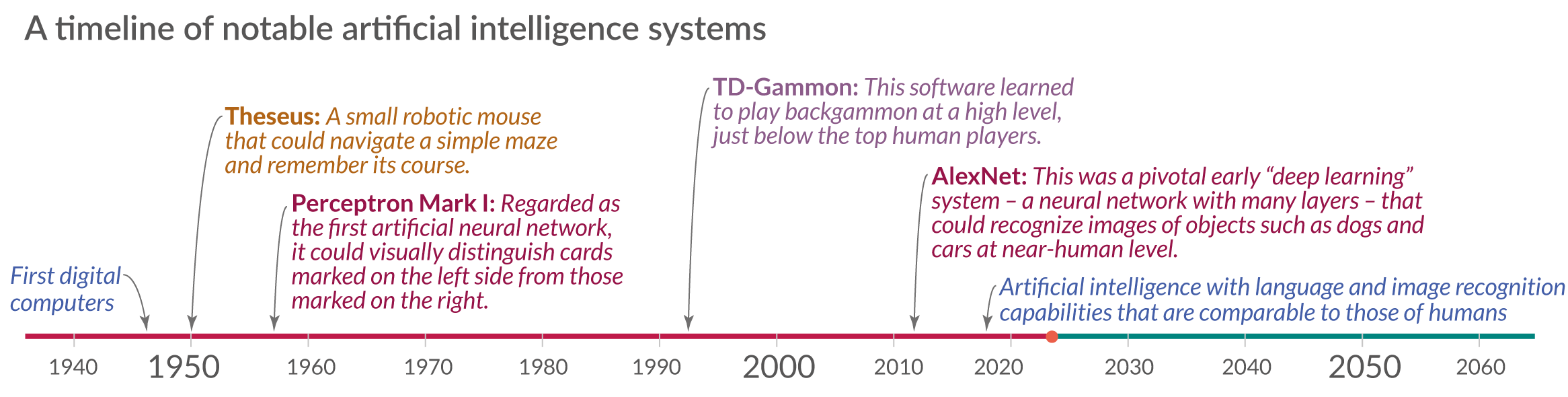
人工智能系统的语言和图像识别能力现在可与人类相媲美。
人工智能系统的语言和图像识别能力发展非常迅速。
该图表通过放大过去二十年的人工智能发展,显示了我们如何走到这一步。绘制的数据源于许多测试,在这些测试中,人类和人工智能的表现在五个不同的领域进行了评估,从手写识别到语言理解。
在五个领域中的每一个域中,AI系统的初始性能都设置为-100,并且这些测试中的人类性能用作设置为零的基线。这意味着,当模型的性能越过零线时,人工智能系统在相关测试中的得分高于进行相同测试的人。2
就在10年前,没有机器能够在人类水平上可靠地提供语言或图像识别。但是,正如图表所示,人工智能系统已经变得越来越强大,现在在所有这些领域的测试中都击败了人类。
在这些标准化测试之外,这些AI的性能参差不齐。在某些现实世界中,这些系统的性能仍然比人类差得多。另一方面,这种人工智能系统的一些实现已经非常便宜,可以在你口袋里的手机上买到:图像识别对你的照片进行分类,语音识别转录你口述的内容。
人工智能系统的语言和图像识别能力迅速提升3
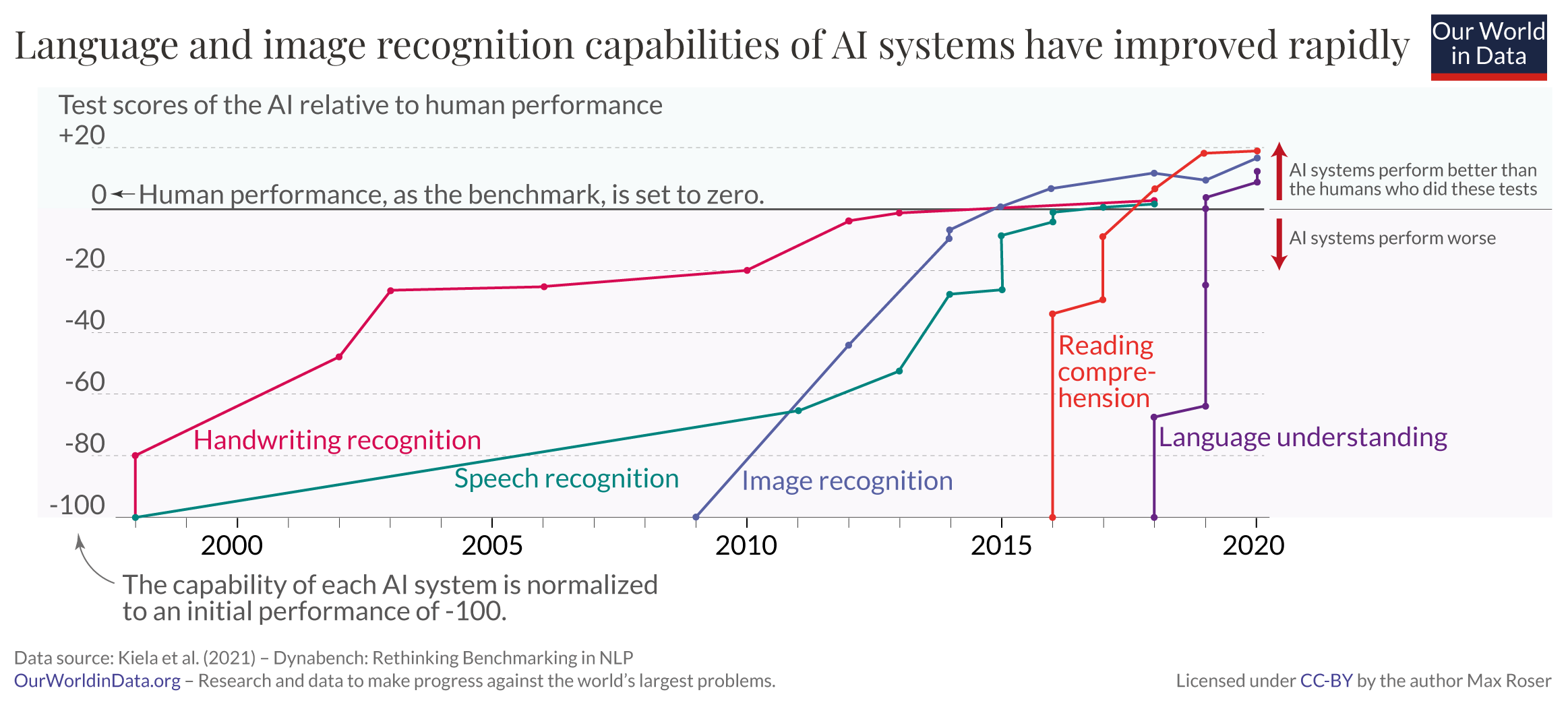
从图像识别到图像生成
上一张图表显示了人工智能感知能力的快速发展。人工智能系统生成图像的能力也大大提高。
这一系列的九张图片显示了过去九年的发展。这些图像中的人都不存在;所有这些都是由人工智能系统生成的。
该系列从左上角的 2014 年图像开始,这是一张黑白像素化脸的原始图像。正如第二行第一张图片所示,仅仅三年后,人工智能系统就已经能够生成难以与照片区分开来的图像。
近年来,人工智能系统的能力变得更加令人印象深刻。虽然早期的系统专注于生成人脸图像,但这些较新的模型将其功能扩展到基于几乎任何提示的文本到图像生成。右下角的图像显示了即使是最具挑战性的提示 - 例如“一只博美犬戴着王冠坐在国王的宝座上。两个老虎士兵站在王座旁边“——在几秒钟内变成逼真的图像。4
人工智能生成的图像的时间轴5
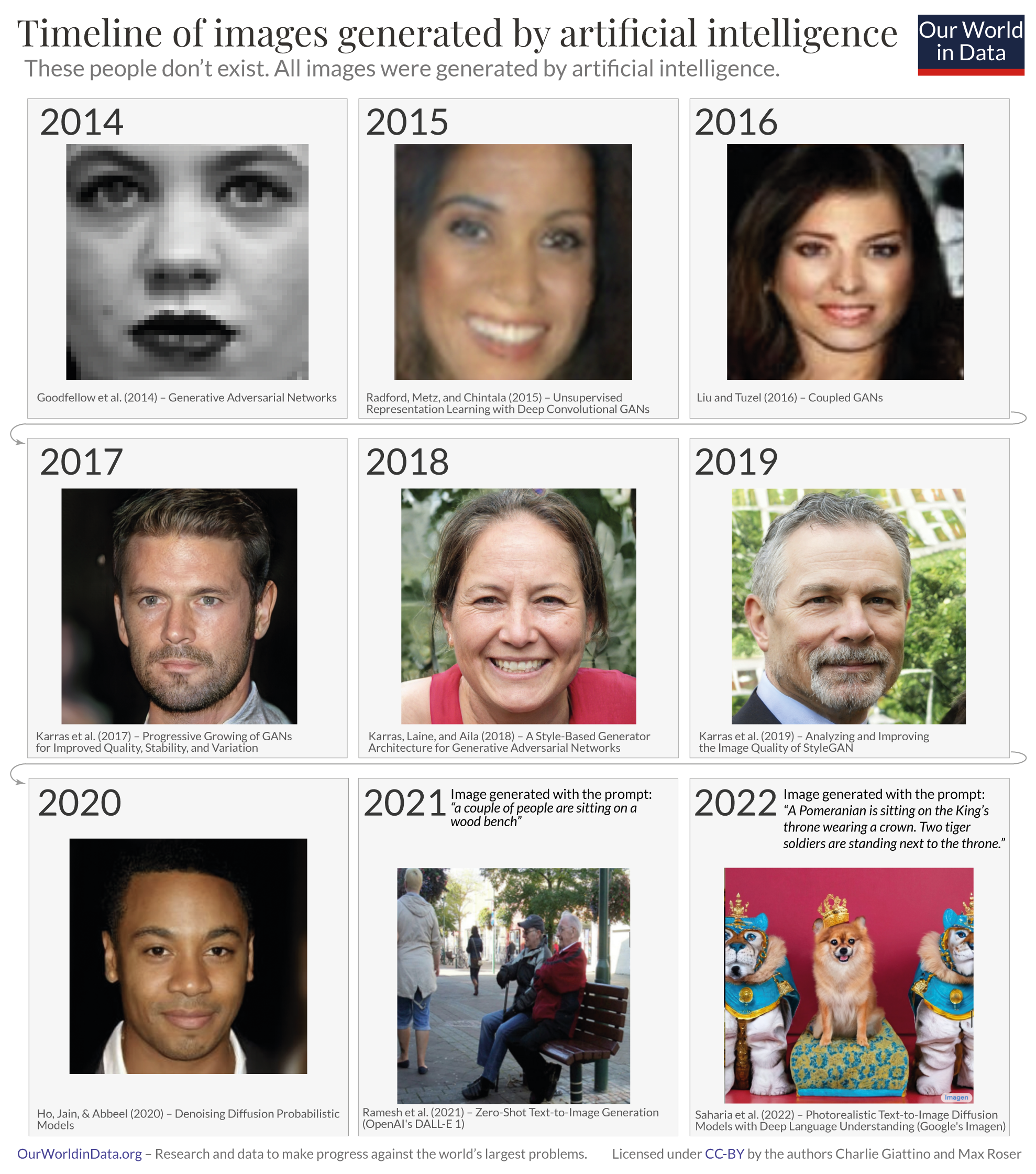
语言识别和生产发展迅速
与图像生成AI的进步同样引人注目的是解析和响应人类语言的系统快速发展。
图中显示了谷歌开发的名为PaLM的AI系统的示例。在这六个例子中,系统被要求解释六个不同的笑话。我发现右下角的解释特别引人注目:人工智能解释了一个专门用来混淆听众的反笑话。
在过去的几年里,产生语言的人工智能以多种方式进入了我们的世界。电子邮件被自动完成,大量的在线文本被翻译,视频被自动转录,学童使用语言模型做作业,报告自动生成,媒体发布人工智能生成的新闻。
人工智能系统还不能产生长而连贯的文本。在未来,我们将看到最近的发展是否会放缓 - 甚至结束 - 或者我们是否有一天会读到人工智能写的畅销小说。
人工智能系统PaLM在被要求解释六个不同的笑话后输出6
我们现在所处的位置:人工智能就在这里
人工智能能力的快速发展使得在广泛的新领域中使用机器成为可能:
当您预订航班时,决定您支付费用的通常是人工智能,而不是人类。当你到达机场时,它是一个人工智能系统,可以监控你在机场做什么。一旦你上了飞机,人工智能系统会协助飞行员将你送到目的地。
人工智能系统也越来越多地决定你是否获得贷款,是否有资格获得福利,或者是否被雇用从事特定工作。他们越来越多地帮助确定谁能从监狱释放。
一些政府正在购买用于战争的自主武器系统,一些政府正在使用人工智能系统进行监视和压迫。
人工智能系统有助于对您使用的软件进行编程并翻译您阅读的文本。在过去十年中,由语音识别操作的虚拟助手已经进入了许多家庭。现在,自动驾驶汽车正在成为现实。
在过去的几年里,人工智能系统帮助在科学中一些最困难的问题上取得了进展。
称为推荐系统的大型AI决定了您在社交媒体上看到的内容,在线商店中向您展示了哪些产品,以及在YouTube上向您推荐了哪些产品。他们越来越多地不仅推荐我们消费的媒体,而且基于他们生成图像和文本的能力,他们还在创造我们消费的媒体。
人工智能不再是未来的技术;人工智能就在这里,现在的现实大部分看起来就像科幻小说一样。这是一项已经影响我们所有人的技术,上面的列表仅包括其众多应用程序中的一小部分。
列出的应用程序范围广泛清楚地表明,这是一项非常通用的技术,人们可以将它用于一些非常好的目标 - 也可以用于一些非常糟糕的目标。对于这种“双重用途技术”,重要的是我们所有人都要了解正在发生的事情以及我们希望如何使用该技术。
就在二十年前,世界还大不相同。人工智能技术在未来可能有什么能力?
下一步是什么?
我们刚刚考虑的人工智能系统是人工智能技术数十年稳步进步的结果。
下面的大图表透视了过去八十年的历史。它基于海梅·塞维利亚及其同事制作的数据集。7
此图表中的每个小圆圈代表一个 AI 系统。圆在水平轴上的位置指示 AI 系统的构建时间,它在纵轴上的位置显示用于训练特定 AI 系统的计算量。
训练计算以浮点运算(简称 FLOP)进行测量。一个 FLOP 相当于两个十进制数的一次加法、减法、乘法或除法。
所有依赖机器学习的人工智能系统都需要训练,在这些系统中,训练计算是驱动系统能力的三个基本因素之一。另外两个因素是用于训练的算法和输入数据。可视化显示,随着训练计算的增加,人工智能系统变得越来越强大。
时间线可以追溯到 1940 年代,即电子计算机的开端。第一个展示的人工智能系统是“忒修斯”,克劳德·香农(Claude Shannon)1950年的机器人鼠标,我在开头提到过。在时间线的另一端,你会发现像DALL-E和PaLM这样的人工智能系统,它们能够产生逼真的图像,解释和生成我们刚刚看到的语言。它们是迄今为止使用最多训练计算的人工智能系统之一。
训练计算绘制在对数刻度上,因此从每条网格线到下一条网格线显示 100 倍的增长。这种长期观点显示出持续增长。在最初的六十年中,训练计算量根据摩尔定律增加,大约每20个月翻一番。自2010年左右以来,这种指数级增长进一步加速,翻了一番,只有大约6个月。这是一个惊人的快速增长速度。8
快速的倍增时间已经累积了大幅增加。PaLM 的训练计算量为 25 亿 petaFLOP,是 10 年前训练计算量最大的 AI AlexNet 的 500 多万倍。9
扩大规模已经呈指数级增长,并且在过去十年中大幅加快。对于人工智能的未来,我们可以从这一历史发展中学到什么?
过去 8 年人工智能的兴起:随着训练计算的增加,人工智能系统变得更加强大10
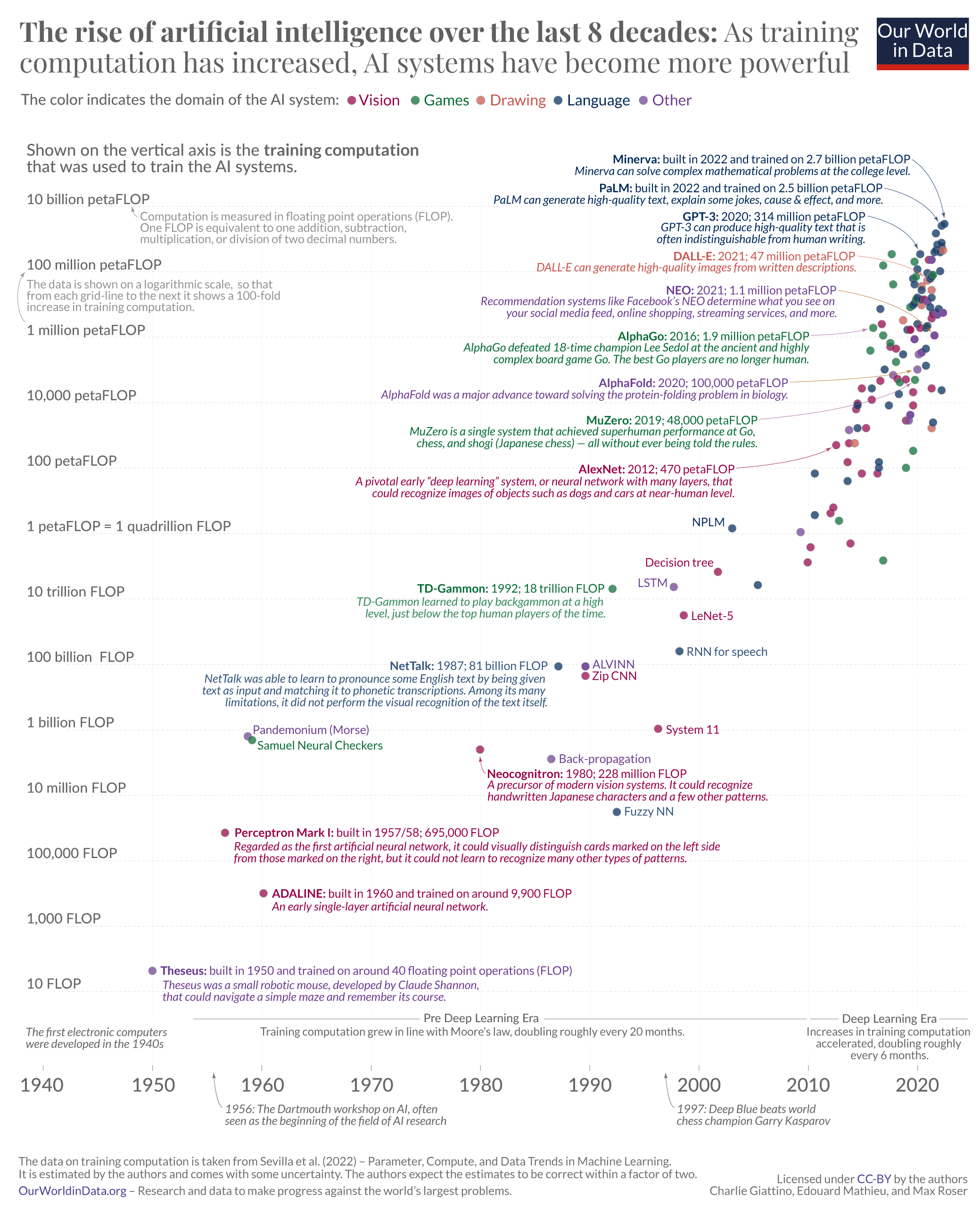
研究长期趋势以预测人工智能的未来
人工智能研究人员研究这些长期趋势,看看未来会发生什么。11
也许最广泛讨论的此类研究是由人工智能研究员Ajeya Cotra发表的。她研究了训练计算的增加,以询问训练人工智能系统的计算在什么时候可以与人脑相匹配。这个想法是,在这一点上,人工智能系统将与人脑的能力相匹配。在她的最新更新中,Cotra估计,到2040年,距离现在不到二十年,这种“变革性人工智能”将被开发出来的可能性为50%。12
在一篇相关文章中,我讨论了变革性的人工智能对世界意味着什么。简而言之,这个想法是这样的人工智能系统将足够强大,可以将世界带入一个“质的不同未来”。它可能导致人类历史上早期两次重大变革——农业革命和工业革命——的规模变化。它肯定是我们一生中最重要的全球变化。
Cotra的工作在这种情况下特别相关,因为她的预测是基于我们刚刚研究的训练计算的历史长期趋势。但值得注意的是,其他依赖不同考虑因素的预测者得出的结论大致相似。正如我在关于人工智能时间表的文章中所展示的那样,许多人工智能专家认为,人类水平的人工智能很有可能在未来几十年内得到发展,有些人认为它会更早地存在。
构建公共资源以实现必要的公共对话
计算机和人工智能极大地改变了我们的世界,但我们仍处于这段历史的早期阶段。因为这项技术感觉如此熟悉,所以很容易忘记我们与之互动的所有这些技术都是最近的创新,而且最深刻的变化尚未到来。
人工智能已经改变了我们所看到的、我们所知道的和我们所做的事情。尽管这项技术只有短暂的历史。
没有迹象表明这些趋势很快就会达到任何极限。相反,特别是在过去十年中,基本趋势加速了:对人工智能技术的投资迅速增加,训练计算的倍增时间缩短到六个月。
所有重大技术创新都会带来一系列积极和消极的后果。人工智能已经如此。随着这项技术变得越来越强大,我们应该期待它的影响会越来越大。
由于人工智能的重要性,我们都应该能够对这项技术的发展方向形成意见,并了解这种发展如何改变我们的世界。为此,我们正在构建一个与 AI 相关的指标存储库,您可以在 OurWorldinData.org/artificial-intelligence 上找到。
我们仍处于这段历史的早期阶段,许多可能发生的事情尚未到来。如此强大的技术发展应该是我们关注的中心。对于我们世界的未来——以及我们生活的未来——将如何发展,可能没有什么比这更重要了。
参考
-
On the Theseus see Daniel Klein (2019) – Mighty mouse, Published in MIT Technology Review. And this video on YouTube of a presentation by its inventor Claude Shannon.
-
The chart shows that the speed with which these AI technologies developed increased over time. Systems for which development was started early – handwriting and speech recognition – took more than a decade to approach human-level performance, while more recent AI developments led to systems that overtook humans in the span of only a few years. However one should not overstate this point. To some extent this is dependent on when the researchers started to compare machine and human performance. One could have started evaluating the system for language understanding much earlier and its development would appear much slower in this presentation of the data.
-
Data from Kiela et al. (2021) – Dynabench: Rethinking Benchmarking in NLP. arXiv:2104.14337v1; https://doi.org/10.48550/arXiv.2104.14337
-
Because these systems have become so powerful, the latest AI systems often don’t allow the user to generate images of human faces to prevent abuse.
-
The relevant publications are the following:
2014: Goodfellow et al: Generative Adversarial Networks
2015: Radford, Metz, and Chintala: Unsupervised Representation Learning with Deep Convolutional Generative Adversarial Networks
2016: Liu and Tuzel: Coupled Generative Adversarial Networks
2017: Karras et al: Progressive Growing of GANs for Improved Quality, Stability, and Variation
2018: Karras, Laine, and Aila: A Style-Based Generator Architecture for Generative Adversarial Networks (StyleGAN from NVIDIA)
2019: Karras et al: Analyzing and Improving the Image Quality of StyleGAN
AI-generated faces generated by this technology can be found on thispersondoesnotexist.com.
2020: Ho, Jain, and Abbeel: Denoising Diffusion Probabilistic Models
2021: Ramesh et al: Zero-Shot Text-to-Image Generation (first DALL-E from OpenAI; blog post). See also Ramesh et al (2022) – Hierarchical Text-Conditional Image Generation with CLIP Latents (DALL-E 2 from OpenAI; blog post).
2022: Saharia et al: Photorealistic Text-to-Image Diffusion Models with Deep Language Understanding (Google’s Imagen; blog post)
-
From Chowdhery et al (2022) – PaLM: Scaling Language Modeling with Pathways. Published on arXiv on 7 Apr 2022.
-
See the footnote on the title of the chart for the references and additional information.
-
At some point in the future, training computation is expected to slow down to the exponential growth rate of Moore’s Law. Tamay Besiroglu, Lennart Heim and Jaime Sevilla of the Epoch team estimate in their report that the highest probability for this reversion occuring is in the early 2030s.
-
The training computation of PaLM, developed in 2022, was 2,700,000,000 petaFLOP. The training computation of AlexNet, the AI with the largest training computation up to 2012, was 470 petaFLOP. 2,500,000,000 petaFLOP / 470 petaFLOP = 5,319,148.9. At the same time the amount of training computation required to achieve a given performance has been falling exponentially.
The costs have also increased quickly. The cost to train PaLM is estimated to be in the range of $9–$23 million according to Lennart Heim, a researcher in the Epoch team. See: Lennart Heim (2022) – Estimating PaLM’s training cost.
-
The data is taken from Jaime Sevilla, Lennart Heim, Anson Ho, Tamay Besiroglu, Marius Hobbhahn, Pablo Villalobos (2022) – Compute Trends Across Three eras of Machine Learning. Published in arXiv on March 9, 2022. See also their post on the Alignment Forum.
The authors regularly update and extend their dataset, a very helpful service to the AI research community. At Our World in Data my colleague Charlie Giattino regularly updates the interactive version of this chart with the latest data made available by Sevilla and coauthors.
See also these two related charts:
Number of parameters in notable artificial intelligence systems
Number of datapoints used to train notable artificial intelligence systems
-
Scaling up the size of neural networks – in terms of the number of parameters and the amount of training data and computation – has led to surprising increases in the capabilities of AI systems. This realization motivated the “scaling hypothesis.” See Gwern Branwen (2020) – The Scaling Hypothesis.
-
Her research was announced in various places, including in the AI Alignment Forum here: Ajeya Cotra (2020) – Draft report on AI timelines. As far as I know the report itself always remained a ‘draft report’ and was published here on Google Docs.
The cited estimate stems from Cotra’s Two-year update on my personal AI timelines, in which she shortened her median timeline by 10 years.
Cotra emphasizes that there are substantial uncertainties around her estimates and therefore communicates her findings in a range of scenarios. She published her big study in 2020 and her median estimate at the time was that around the year 2050 there will be a 50%-probability that the computation required to train such a model may become affordable. In her “most conservative plausible”-scenario this point in time is pushed back to around the year 2090 and in her “most aggressive plausible”-scenario this point in time is reached in 2040.
The same is true for most other forecasters: all emphasize the large uncertainty associated with any of their forecasts.
It is worth emphasizing that the computation of the human brain is highly uncertain. See Joseph Carlsmith’s New Report on How Much Computational Power It Takes to Match the Human Brain from 2020.
内容中包含的图片若涉及版权问题,请及时与我们联系删除
评论
沙发等你来抢