vLLM 是伯克利大学 LMSYS 组织开源的大语言模型高速推理框架,极大地提升了实时场景下的 LLM 服务的吞吐与内存使用效率。vLLM 主要采用了 Paged Attention 技术,结合 Continuous Batching,对传入请求进行持续分批处理,同时优化了CUDA内核。
vLLM 的灵活性和易用性可以使得开发者构建高吞吐量的大语言模型服务,支持流式输出,支持单机多卡、分布式并行推理
vLLM 官方现已经支持 Aquila-7B模型 。经过实测,AquilaChat-7B 使用 vLLM 推理速度相比 HuggingFace Transfomers 提升了 54%。
举例: 输入“请给出10个要到北京旅游的理由。” 推理过程参数如下:
推理方式 | 速度 | 内存 | 参数 |
HuggingFace Transformers | 30.11 tokens/秒 | 29.22 GB | Max length 1000 |
vllm | 65.06 tokens/秒 | 34.04 GB | Max length 1000 |
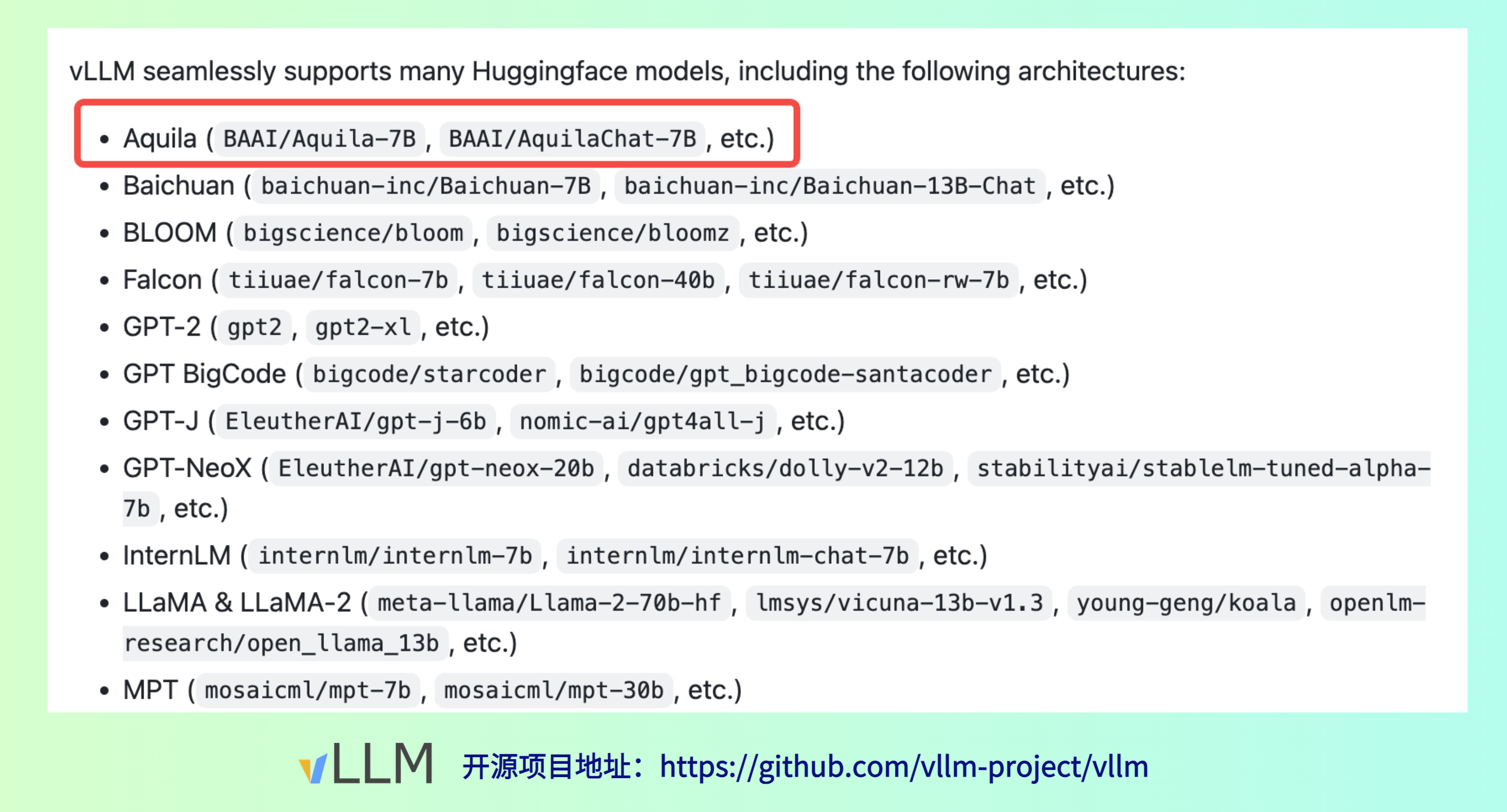
操作步骤
第一步 安装依赖
配置需求
- OS: Linux
- Python: 3.8 or higher
- CUDA: 11.0 – 11.8
- GPU: compute capability 7.0 or higher (e.g., V100, T4, RTX20xx, A100, L4, etc.)
安装vllm
pip install vllm # This may take 5-10 minutes.
第二步 模型文件准备
- 模型权重
- 如果使用Aquila模型,通过 Hugging Face 下载 Aquila 系列模型 https://huggingface.co/BAAI;
- 如果基于FlagAI微调后,需要通过转换脚本 将权重转换为Hugging Face模型权重格式。
- 模型Tokenizer保持不变
- tokenizer_config.json
- tokenizer.json
- vocab.json
- merges.txt
第三步 使用vllm推理
from vllm import LLM, SamplingParams
prompts = ['去北京的10个理由。',]
sampling_params = SamplingParams(temperature=1, top_p=0.9,top_k=50, max_tokens=100, stop="")
llm = LLM(model="BAAI/AquilaChat-7B", tensor_parallel_size=1, trust_remote_code=True)
outputs = llm.generate(prompts, sampling_params)# Print the outputs.
for output in outputs:
prompt = output.prompt
generated_text = output.outputs[0].text
print(f"Prompt: {prompt!r}, Generated text: {generated_text!r}")
悟道·天鹰Aquila系列模型是智源研究院推出的开源大语言模型,支持免费商用许可。
使用方式一(推荐):通过 FlagAI 加载 Aquila 系列模型 https://github.com/FlagAI-Open/FlagAI/tree/master/examples/Aquila
使用方式二:通过 FlagOpen 模型仓库单独下载权重 https://model.baai.ac.cn/
使用方式三:通过 Hugging Face 加载 Aquila 系列模型 https://huggingface.co/BAAI
内容中包含的图片若涉及版权问题,请及时与我们联系删除
评论
沙发等你来抢