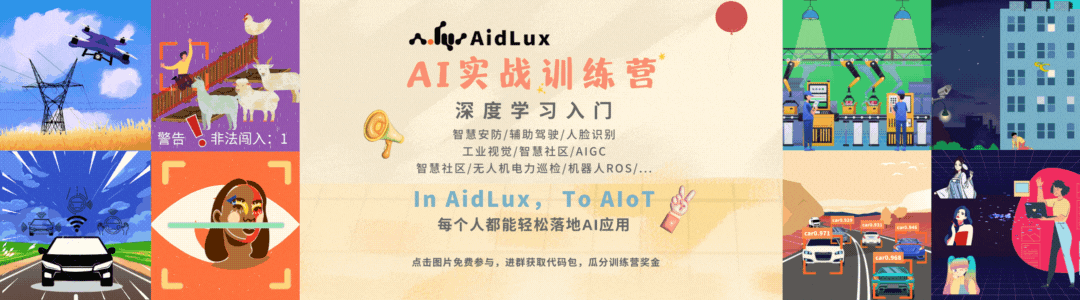
以下文章来源于知乎:小白学视觉
作者:小白学视觉
链接:https://mp.weixin.qq.com/s/piciiiapXRDsDRx7-VdiCg
本文仅用于学术分享,如有侵权,请联系后台作删文处理

背景介绍
疲劳驾驶的危害不堪设想,据了解,21%的交通事故都因此而生,尤其是高速路上,大多数车辆都是长途驾驶,加之速度快,危害更加严重。
实现步骤
思路:疲劳驾驶的司机大部分都有打瞌睡的情形,所以我们根据驾驶员眼睛闭合的频率和时间来判断驾驶员是否疲劳驾驶(或嗜睡)。
详细实现步骤
【1】眼部关键点检测。
关于MediaPipe前面已经介绍过,具体可以查看下面链接的文章:
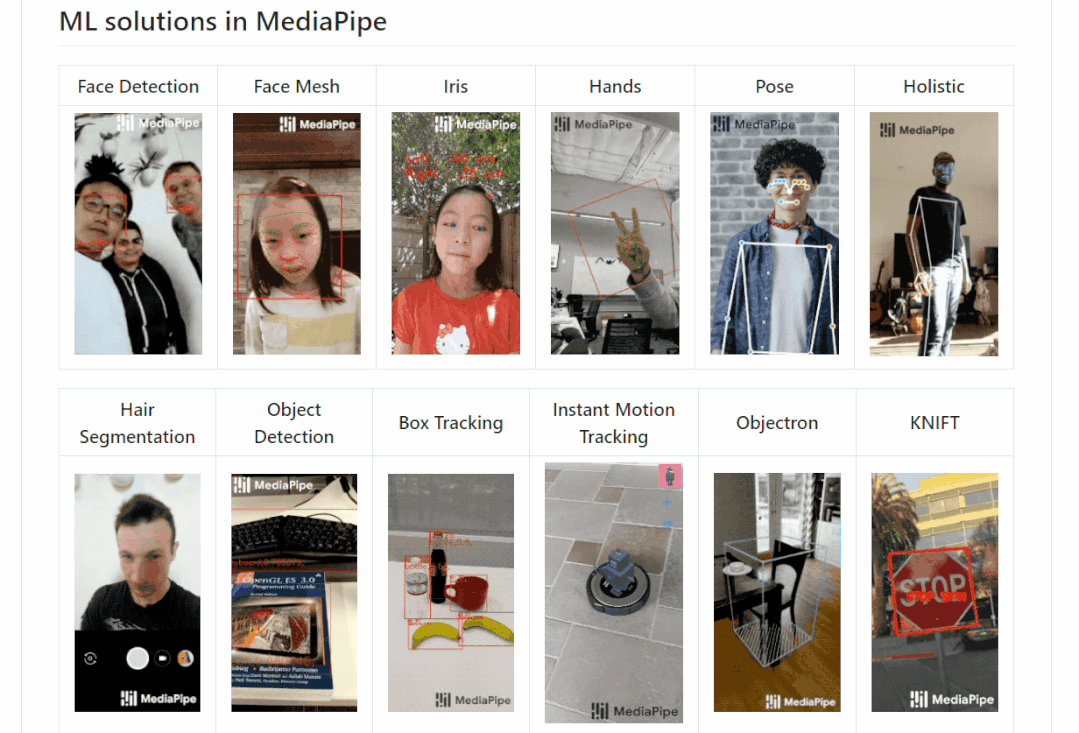
我们使用Face Mesh来检测眼部关键点,Face Mesh返回了468个人脸关键点:
对于左眼: [362, 385, 387, 263, 373, 380]
对于右眼:[33, 160, 158, 133, 153, 144]
import cv2
import numpy as np
import matplotlib.pyplot as plt
import mediapipe as mp
mp_facemesh = mp.solutions.face_mesh
mp_drawing = mp.solutions.drawing_utils
denormalize_coordinates = mp_drawing._normalized_to_pixel_coordinates
%matplotlib inline
# Landmark points corresponding to left eye
all_left_eye_idxs = list(mp_facemesh.FACEMESH_LEFT_EYE)
# flatten and remove duplicates
all_left_eye_idxs = set(np.ravel(all_left_eye_idxs))
# Landmark points corresponding to right eye
all_right_eye_idxs = list(mp_facemesh.FACEMESH_RIGHT_EYE)
all_right_eye_idxs = set(np.ravel(all_right_eye_idxs))
# Combined for plotting - Landmark points for both eye
all_idxs = all_left_eye_idxs.union(all_right_eye_idxs)
# The chosen 12 points: P1, P2, P3, P4, P5, P6
chosen_left_eye_idxs = [362, 385, 387, 263, 373, 380]
chosen_right_eye_idxs = [33, 160, 158, 133, 153, 144]
all_chosen_idxs = chosen_left_eye_idxs + chosen_right_eye_idx
【2】检测眼睛是否闭合——计算眼睛纵横比(EAR)。
要检测眼睛是否闭合,我们可以使用眼睛纵横比(EAR) 公式:
EAR 公式返回反映睁眼程度的单个标量:
1. 我们将使用 Mediapipe 的 Face Mesh 解决方案来检测和检索眼睛区域中的相关地标(下图中的点P 1 - P 6)。
2. 检索相关点后,会在眼睛的高度和宽度之间计算眼睛纵横比 (EAR)。
当眼睛睁开并接近零时,EAR 几乎是恒定的,而闭上眼睛是部分人,并且头部姿势不敏感。睁眼的纵横比在个体之间具有很小的差异。它对于图像的统一缩放和面部的平面内旋转是完全不变的。由于双眼同时眨眼,所以双眼的EAR是平均的。
def distance(point_1, point_2):
"""Calculate l2-norm between two points"""
dist = sum([(i - j) ** 2 for i, j in zip(point_1, point_2)]) ** 0.5
return dist
def get_ear(landmarks, refer_idxs, frame_width, frame_height):
"""
Calculate Eye Aspect Ratio for one eye.
Args:
landmarks: (list) Detected landmarks list
refer_idxs: (list) Index positions of the chosen landmarks
in order P1, P2, P3, P4, P5, P6
frame_width: (int) Width of captured frame
frame_height: (int) Height of captured frame
Returns:
ear: (float) Eye aspect ratio
"""
try:
# Compute the euclidean distance between the horizontal
coords_points = []
for i in refer_idxs:
lm = landmarks[i]
coord = denormalize_coordinates(lm.x, lm.y,
frame_height)
coords_points.append(coord)
# Eye landmark (x, y)-coordinates
P2_P6 = distance(coords_points[1], coords_points[5])
P3_P5 = distance(coords_points[2], coords_points[4])
P1_P4 = distance(coords_points[0], coords_points[3])
# Compute the eye aspect ratio
ear = (P2_P6 + P3_P5) / (2.0 * P1_P4)
except:
ear = 0.0
coords_points = None
return ear, coords_points
def calculate_avg_ear(landmarks, left_eye_idxs, right_eye_idxs, image_w, image_h):
"""Calculate Eye aspect ratio"""
left_ear, left_lm_coordinates = get_ear(
landmarks,
left_eye_idxs,
image_w,
image_h
)
right_ear, right_lm_coordinates = get_ear(
landmarks,
right_eye_idxs,
image_w,
image_h
)
Avg_EAR = (left_ear + right_ear) / 2.0
return Avg_EAR, (left_lm_coordinates, right_lm_coordinates)
image_eyes_open = cv2.imread("test-open-eyes.jpg")[:, :, ::-1]
image_eyes_close = cv2.imread("test-close-eyes.jpg")[:, :, ::-1]
for idx, image in enumerate([image_eyes_open, image_eyes_close]):
image = np.ascontiguousarray(image)
imgW, _ = image.shape
# Creating a copy of the original image for plotting the EAR value
custom_chosen_lmk_image = image.copy()
# Running inference using static_image_mode
with mp_facemesh.FaceMesh(refine_landmarks=True) as face_mesh:
results = face_mesh.process(image).multi_face_landmarks
# If detections are available.
if results:
for face_id, face_landmarks in enumerate(results):
landmarks = face_landmarks.landmark
_ = calculate_avg_ear(
imgH
)
# Print the EAR value on the custom_chosen_lmk_image.
{round(EAR, 2)}", (1, 24), :
(255, 255, 255), 2
image.copy(), =
img_eye_lmks_chosen=custom_chosen_lmk_image,
face_landmarks=face_landmarks,
ts_thickness=1,
ts_circle_radius=3,
lmk_circle_radius=3
)
首先,我们声明两个阈值和一个计数器。
EAR_thresh: 用于检查当前EAR值是否在范围内的阈值。
D_TIME:一个计数器变量,用于跟踪当前经过的时间量EAR < EAR_THRESH.
WAIT_TIME:确定经过的时间量是否EAR < EAR_THRESH超过了允许的限制。
当应用程序启动时,我们将当前时间(以秒为单位)记录在一个变量中t1并读取传入的帧。
接下来,我们预处理并frame通过Mediapipe 的 Face Mesh 解决方案管道。
如果有任何地标检测可用,我们将检索相关的 ( Pi )眼睛地标。否则,在此处重置t1 和重置以使算法一致)。D_TIME (D_TIME
如果检测可用,则使用检索到的眼睛标志计算双眼的平均EAR值。
如果是当前时间,则加上当前时间和to之间的差。然后将下一帧重置为。EAR < EAR_THRESHt2t1D_TIMEt1 t2
如果D_TIME >= WAIT_TIME,我们会发出警报或继续下一帧。
推荐阅读
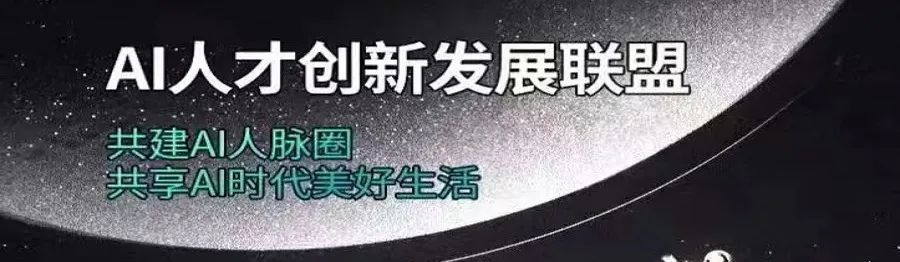
AIHIA | AI人才创新发展联盟2023年盟友招募
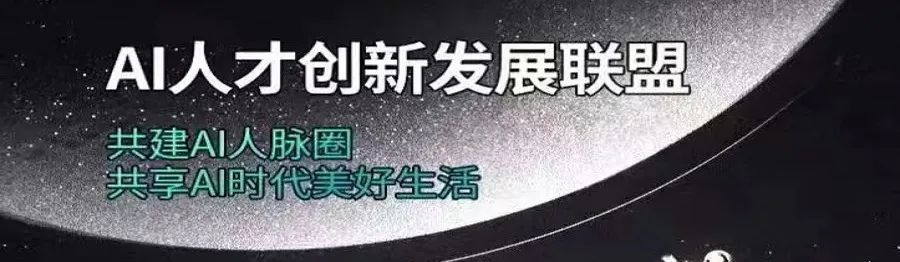
AIHIA | AI人才创新发展联盟2023年盟友招募
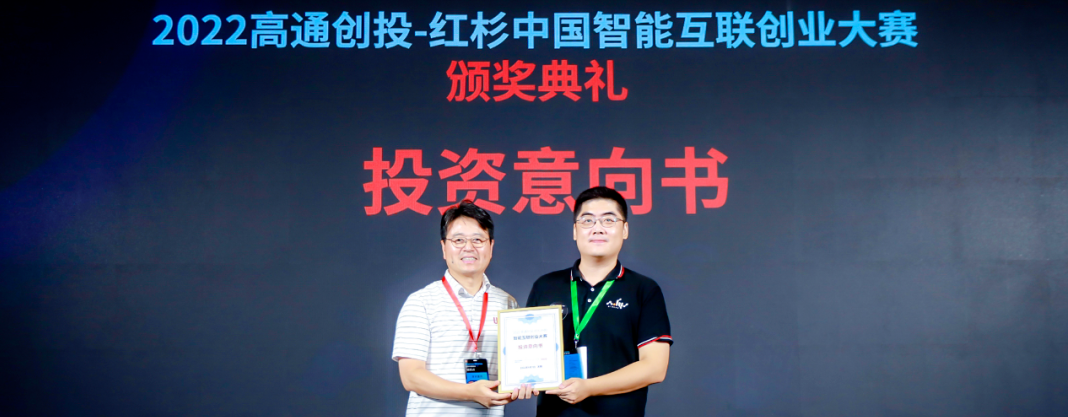
AI融资 | 智能物联网公司阿加犀获得高通5000W融资
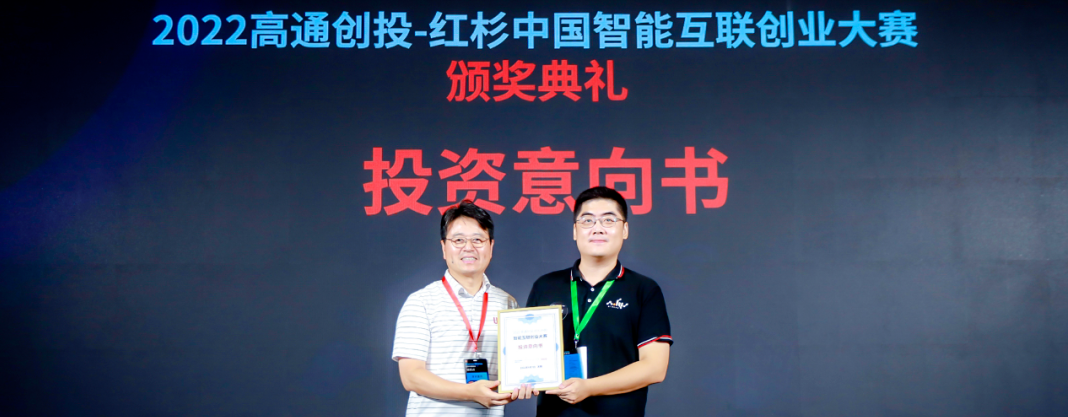
AI融资 | 智能物联网公司阿加犀获得高通5000W融资
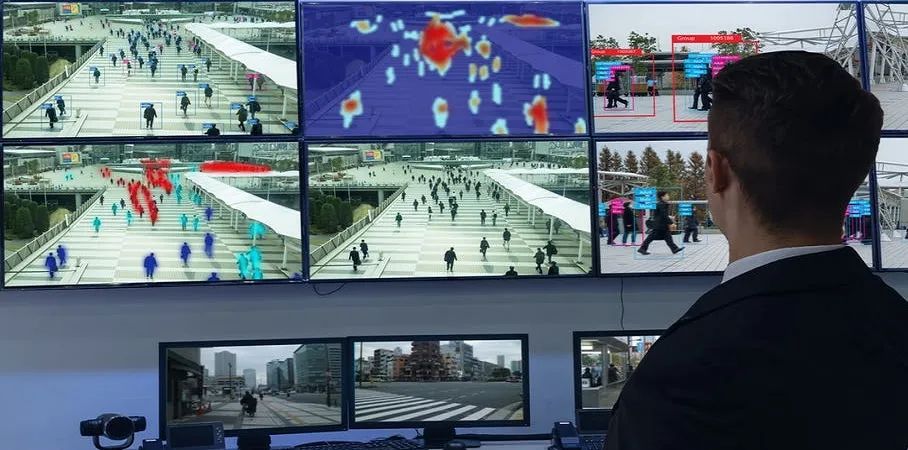
Yolov5应用 | 家庭安防告警系统全流程及代码讲解
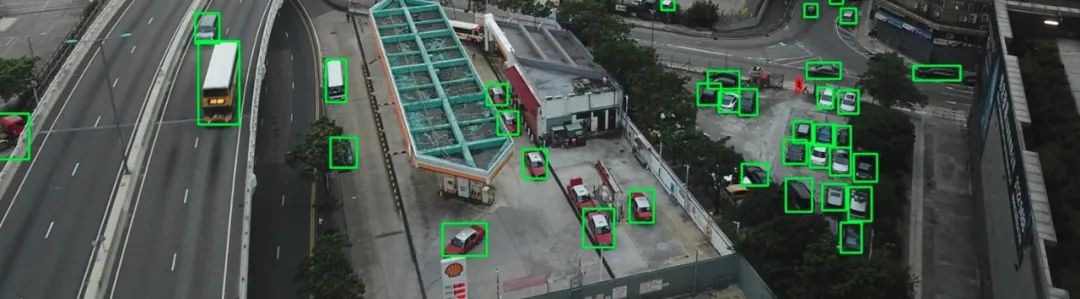
江大白 | 这些年从0转行AI行业的一些感悟
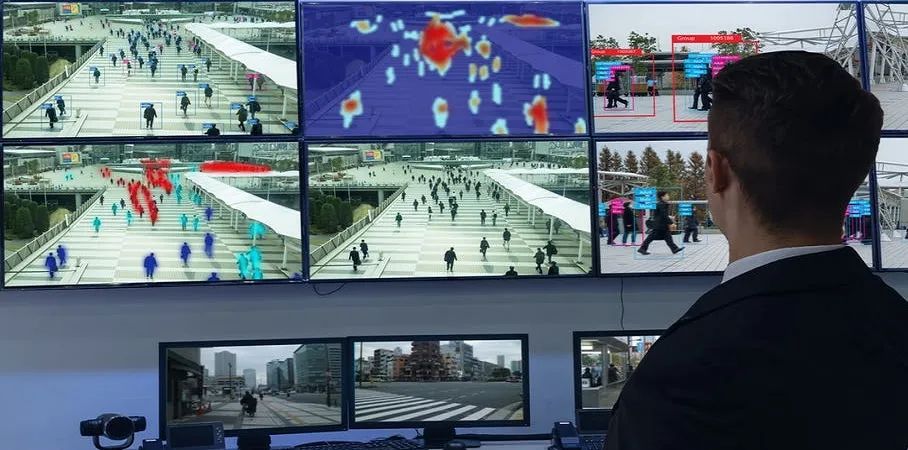
Yolov5应用 | 家庭安防告警系统全流程及代码讲解
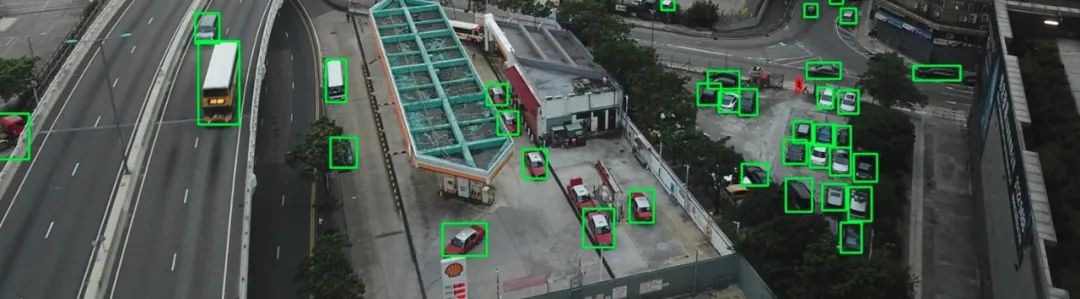
江大白 | 这些年从0转行AI行业的一些感悟
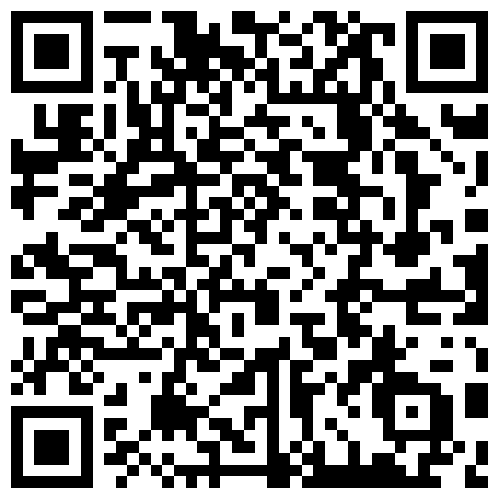
《AI未来星球》陪伴你在AI行业成长的社群,各项福利重磅开放:
(1)198元《31节课入门人工智能》视频课程;
(2)大白花费近万元购买的各类数据集;
(3)每月自习活动,每月17日星球会员日,各类奖品送不停;
(4)加入《AI未来星球》内部微信群;
还有各类直播时分享的文件、研究报告,一起扫码加入吧!
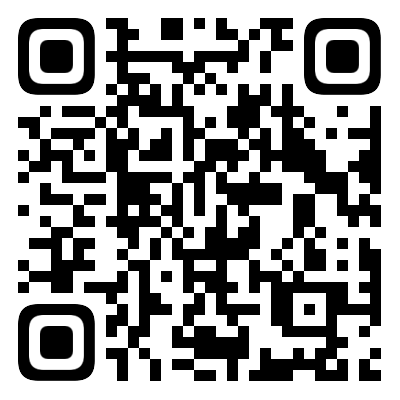
大家一起加油!
内容中包含的图片若涉及版权问题,请及时与我们联系删除
评论
沙发等你来抢