导语

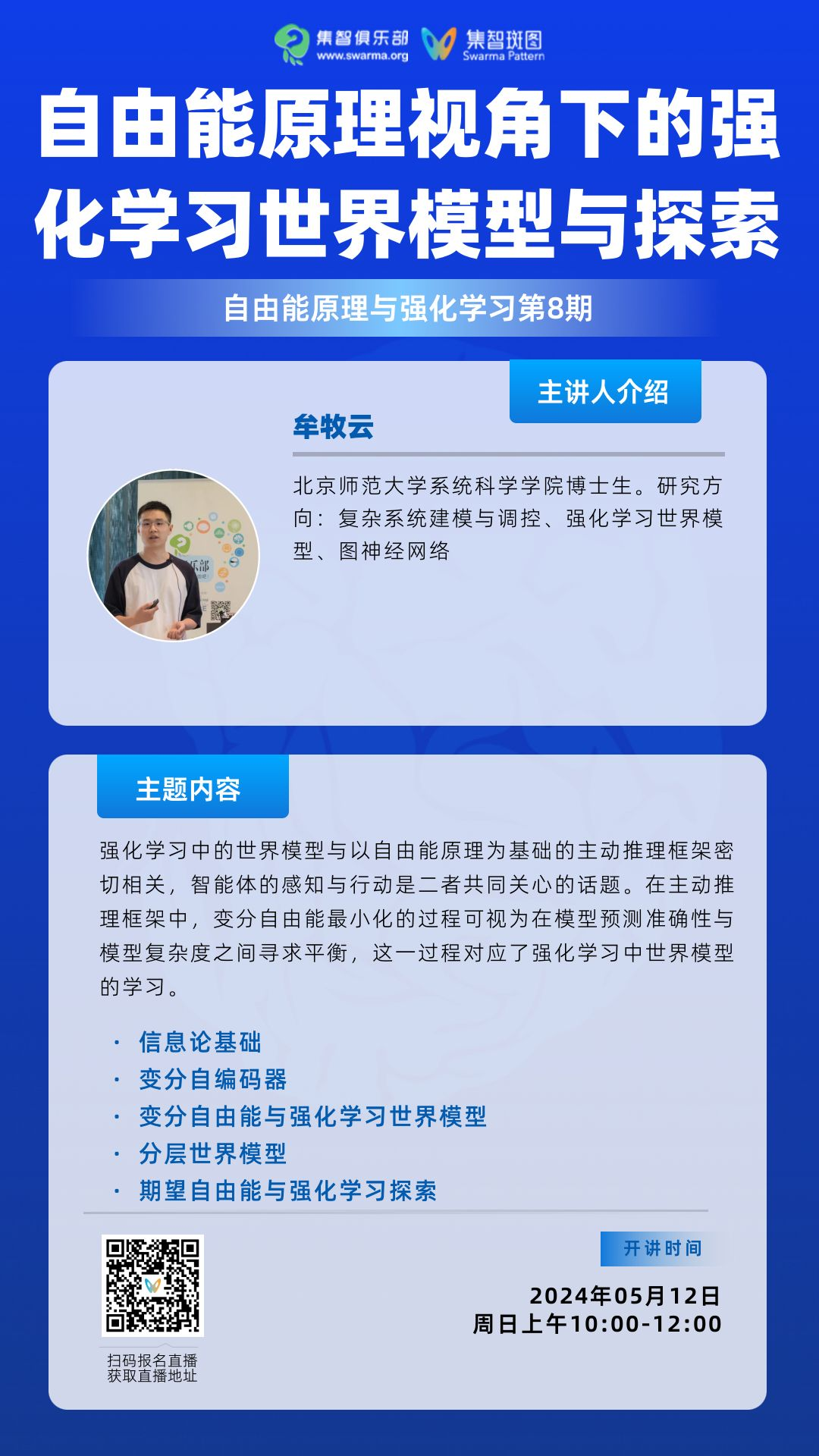
内容简介
内容简介
内容大纲
内容大纲
信息论基础
变分自编码器
变分自由能与强化学习世界模型
分层世界模型
期望自由能与强化学习探索
关键词
关键词
世界模型 World Model
强化学习探索 Reinforcement Learning Exploration
变分自编码器 Variational autoencoder
互信息 Mutual Information
信息增益 Information Gain
参考文献
参考文献
Pinkard H, Waller L. A visual introduction to information theory[J]. arXiv preprint arXiv:2206.07867, 2022.
Higgins I, Matthey L, Pal A, et al. beta-vae: Learning basic visual concepts with a constrained variational framework[J]. 2016.
Ha D, Schmidhuber J. Recurrent world models facilitate policy evolution[J]. Advances in neural information processing systems, 2018, 31.
Mazzaglia P, Verbelen T, Çatal O, et al. The free energy principle for perception and action: A deep learning perspective[J]. Entropy, 2022, 24(2): 301.
Hafner D, Lillicrap T, Fischer I, et al. Learning latent dynamics for planning from pixels. ICML 2019
Hafner D, Ortega P A, Ba J, et al. Action and perception as divergence minimization[J]. arXiv preprint arXiv:2009.01791, 2020.
Hafner D, Lillicrap T, Ba J, et al. Dream to control: Learning behaviors by latent imagination[J]. arXiv preprint arXiv:1912.01603, 2019.
Hafner D, Lillicrap T, Norouzi M, et al. Mastering atari with discrete world models[J]. arXiv preprint arXiv:2010.02193, 2020.
Hafner D, Pasukonis J, Ba J, et al. Mastering diverse domains through world models[J]. arXiv preprint arXiv:2301.04104, 2023.
Saxena V, Ba J, Hafner D. Clockwork variational autoencoders[J]. NIPS 2021, 34: 29246-29257.
Gumbsch C, Sajid N, Martius G, et al. Learning Hierarchical World Models with Adaptive Temporal Abstractions from Discrete Latent Dynamics. ICLR 2024
Hao J, Yang T, Tang H, et al. Exploration in deep reinforcement learning: From single-agent to multiagent domain[J]. IEEE Transactions on Neural Networks and Learning Systems, 2023.
Pathak D, Gandhi D, Gupta A. Self-supervised exploration via disagreement. ICML 2019
Sekar R, Rybkin O, Daniilidis K, et al. Planning to explore via self-supervised world models. ICML 2020
Shyam P, Jaśkowski W, Gomez F. Model-based active exploration. ICML, 2019
Houthooft R, Chen X, Duan Y, et al. Vime: Variational information maximizing exploration. NIPS 2016
Mazzaglia P, Catal O, Verbelen T, et al. Curiosity-driven exploration via latent bayesian surprise. AAAI 2022
主讲人
主讲人
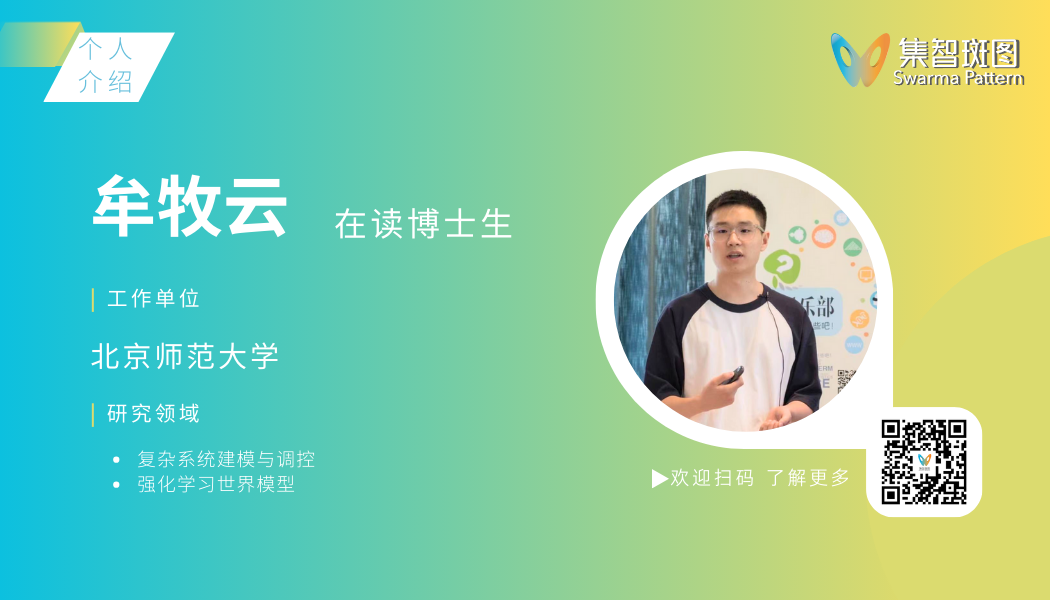
参与方式
参与方式
时间:2024年5月12日(本周日)上午10:00-12:00
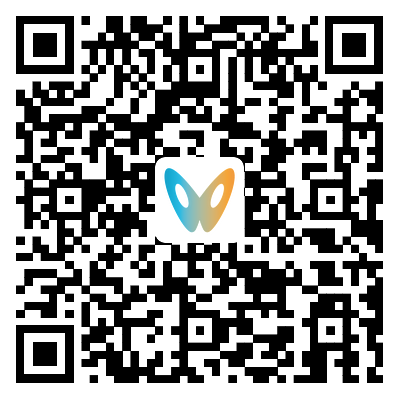
斑图地址:https://pattern.swarma.org/study_group_issue/629
自由能原理与强化学习读书会招募中
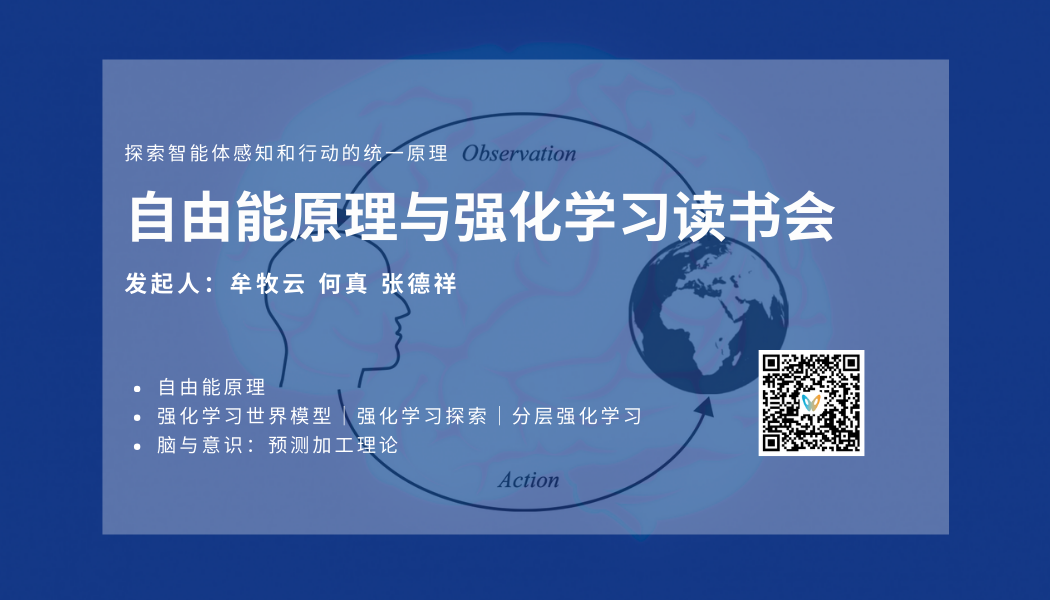
点击“阅读原文”,报名读书会
内容中包含的图片若涉及版权问题,请及时与我们联系删除
评论
沙发等你来抢