Datawhale干货
作者:邹雨衡,Datawhale成员
Datawhale干货
作者:邹雨衡,Datawhale成员
智谱于 2024年6月5日发布了其新一代开源模型——GLM-4-9B,以 9B 的体量,同时支持了 128K 长上下文推理、26种语言多语言支持,且在多个经典评测任务上都实现了超过以往同等开源模型的效果。在 GLM-4-9B 发布之初,我们抢先体验了其效果,并在多种开发者常见任务上进行评测,帮助开发者进一步了解该模型的使用和性能。
接口使用
智谱此次新推出的新模型 GLM-4-9B 的 API 和之前模型接口保持了一致的调用方式,只需将调用的模型切换为新版名称即可。我们可以基于文档给出的接口示例,定义一个简单的函数来获取模型输出:
import time
from zhipuai import ZhipuAI
def get_completion(prompt:str, history=None):
client = ZhipuAI(api_key="your api key") # 请填写您自己的APIKey
if history is None:
history = []
history.append({"role":"user", "content":prompt})
response = client.chat.asyncCompletions.create(
model="glm-4-9b", # 填写最新的模型
messages=history,
)
task_id = response.id
task_status = ''
get_cnt = 0
while task_status != 'SUCCESS' and task_status != 'FAILED' and get_cnt <= 40:
result_response = client.chat.asyncCompletions.retrieve_completion_result(id=task_id)
task_status = result_response.task_status
time.sleep(2)
get_cnt += 1
return result_response.choices[0].message.content
整体使用上来说,API 响应速度较快,明显优于其他厂商的同类模型;接口调用方式也并不复杂,但是和主流的 request 访问方式有一些区别,初级开发者需要在示例代码基础上做一些小改动来适配自己的应用。
在之后的测评中,我们都会使用该函数来调用智谱的新模型;我们分别选取了国内外两个顶尖性能的模型接口 GPT-4 和 讯飞星火大模型v3.5 来进行横向对比,以分析该模型更适合在哪个场景下使用。调用 GPT-4 与讯飞星火大模型的代码此处就不一一展示了,在下文中我们会通过 get_compeletion 函数调用我们此次测评的主角——GLM-4-9B 模型,通过 get_completion_gpt 函数调用 GPT-4,并通过 get_completion_spark 调用讯飞星火大模型。
通用能力测评
接下来我们将分别从多个日常高频使用场景的方面来对此次的新模型进行评测。
首先是最基本的自我认知——你是谁:

To effectively leverage our pretraining data in Llama 3 models, we put substantial effort into scaling up pretraining. Specifically, we have developed a series of detailed scaling laws for downstream benchmark evaluations. These scaling laws enable us to select an optimal data mix and to make informed decisions on how to best use our training compute. Importantly, scaling laws allow us to predict the performance of our largest models on key tasks (for example, code generation as evaluated on the HumanEval benchmark—see above) before we actually train the models. This helps us ensure strong performance of our final models across a variety of use cases and capabilities.
We made several new observations on scaling behavior during the development of Llama 3. For example, while the Chinchilla-optimal amount of training compute for an 8B parameter model corresponds to ~200B tokens, we found that model performance continues to improve even after the model is trained on two orders of magnitude more data. Both our 8B and 70B parameter models continued to improve log-linearly after we trained them on up to 15T tokens. Larger models can match the performance of these smaller models with less training compute, but smaller models are generally preferred because they are much more efficient during inference.
To train our largest Llama 3 models, we combined three types of parallelization: data parallelization, model parallelization, and pipeline parallelization. Our most efficient implementation achieves a compute utilization of over 400 TFLOPS per GPU when trained on 16K GPUs simultaneously. We performed training runs on two custom-built 24K GPU clusters. To maximize GPU uptime, we developed an advanced new training stack that automates error detection, handling, and maintenance. We also greatly improved our hardware reliability and detection mechanisms for silent data corruption, and we developed new scalable storage systems that reduce overheads of checkpointing and rollback. Those improvements resulted in an overall effective training time of more than 95%. Combined, these improvements increased the efficiency of Llama 3 training by ~three times compared to Llama 2.
GLM4-9B 给出的翻译为: 我们用红线划出了翻译的不准确或是不符合中文使用习惯的地方,可以看到,模型对深度学习领域的专业术语翻译都基本准确,略有几处翻译不符合中文使用习惯,但用于辅助论文阅读绰绰有余。 GPT-4 给出的翻译为: 可以看到,GPT-4 对一些专业术语的翻译出现了较大的偏差,如流水线并行化、扩展定律等;同时,GPT-4 给出的翻译结果不符合中文使用习惯的现象明显更严重,且出现了一些病句(如缺少主语、情感倾向不当等)。 星火大模型给出的翻译为: 星火和 GPT-4 的翻译结果类似。
场景二:创新文案编辑
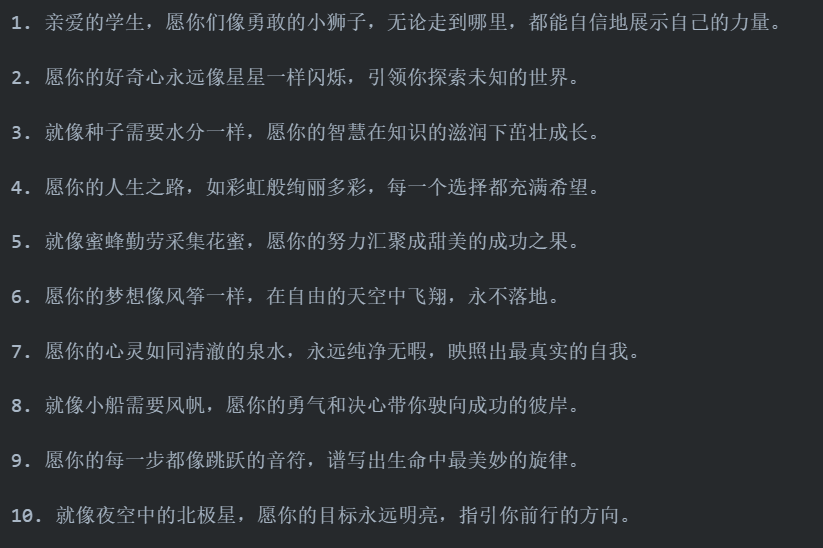
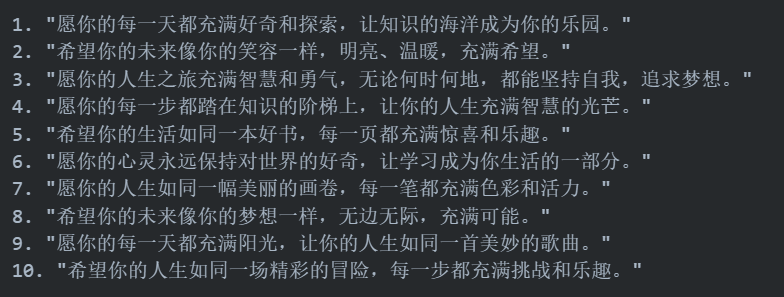
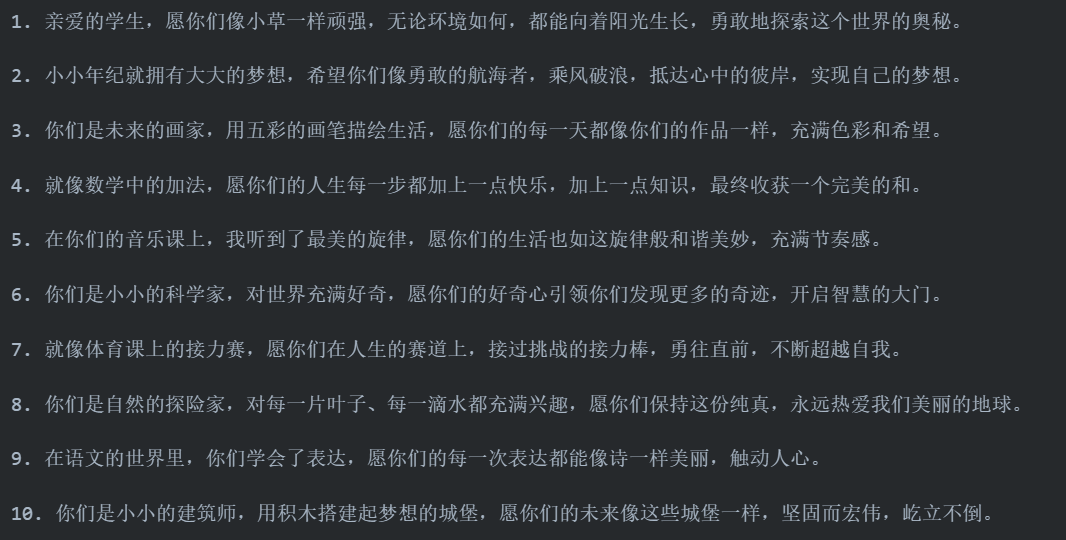
场景三:代码生成
class Solution:
def maximumPrimeDifference(self, nums: List[int]) ->int:
ans = 0
lastp = -1
for x in nums:
if self.isprime(x):
if lastp != -1:
ans = max(ans, x - lastp)
lastp = x
return ans
def isprime(self, x: int) ->bool:
if x < 2:
return False
for i in range(2, int(x ** 0.5) + 1):
if x % i == 0:
return False
return True
class Solution:
def maximumPrimeDifference(self, nums: List[int]) -> int:
def is_prime(n):
if n < 2:
return False
for i in range(2, int(n**0.5) + 1):
if n % i == 0:
return False
return True
min_prime_index = float('inf')
max_prime_index = float('-inf')
for i, num in enumerate(nums):
if is_prime(num):
min_prime_index = min(min_prime_index, i)
max_prime_index = max(max_prime_index, i)
return max_prime_index - min_prime_index
class Solution:
def maximumPrimeDifference(self, nums: List[int]) ->int:
ans = 0
lastp = -1
for x in nums:
if self.isprime(x):
if lastp != -1:
ans = max(ans, x - lastp)
lastp = x
return ans
def isprime(self, x: int) ->bool:
if x < 2:
return False
for i in range(2, int(x ** 0.5) + 1):
if x % i == 0:
return False
return True
场景四:代码解释
def process_func(example):
MAX_LENGTH = 384
input_ids, attention_mask, labels = [], [], []
instruction = tokenizer(f"<|im_start|>system\n现在你要扮演皇帝身边的女人--甄嬛<|im_end|>\n<|im_start|>user\n{example['instruction'] + example['input']}<|im_end|>\n<|im_start|>assistant\n", add_special_tokens=False)
response = tokenizer(f"{example['output']}", add_special_tokens=False)
input_ids = instruction["input_ids"] + response["input_ids"] + [tokenizer.pad_token_id]
attention_mask = instruction["attention_mask"] + response["attention_mask"] + [1]
labels = [-100] * len(instruction["input_ids"]) + response["input_ids"] + [tokenizer.pad_token_id]
if len(input_ids) > MAX_LENGTH:
input_ids = input_ids[:MAX_LENGTH]
attention_mask = attention_mask[:MAX_LENGTH]
labels = labels[:MAX_LENGTH]
return {
"input_ids": input_ids,
"attention_mask": attention_mask,
"labels": labels
}
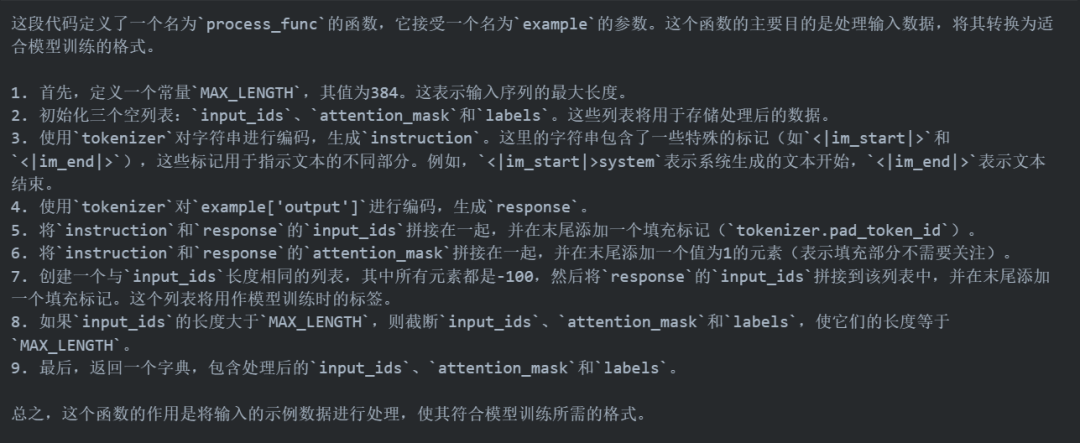
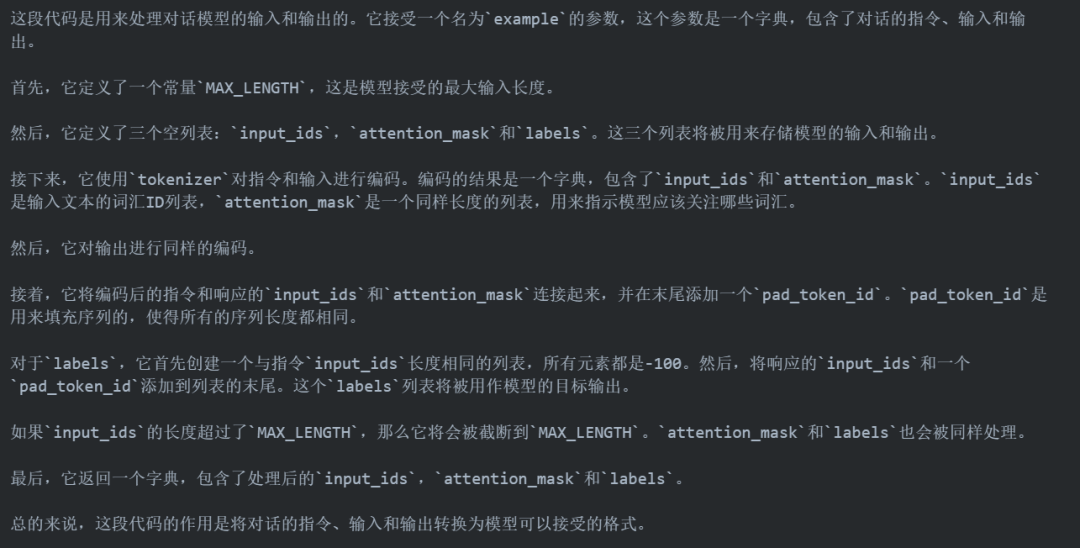
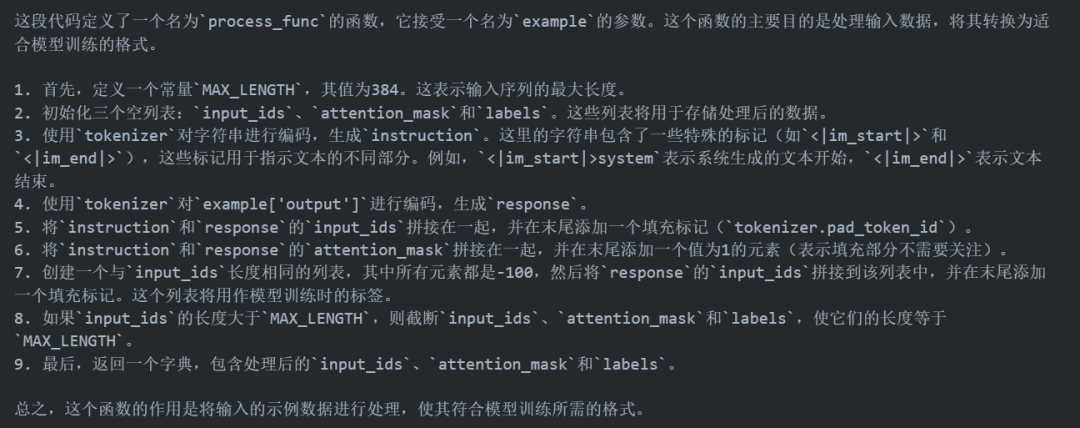
有一个很古老的村子,这个村子的人分两种,红眼睛和蓝眼睛,这两种人并没有什么不同,小孩在没生出来之前,没人知道他是什么颜色的眼睛,这个村子中间有一个广场,是村民们聚集的地方,现在这个村子只有三个人,分住三处。 在这个村子,有一个规定,就是如果一个人能知道自己眼睛的颜色并且在晚上自杀的话,他就会升入天堂,这三个人不能够用语言告诉对方眼睛的颜色,也不能用任何方式提示对方的眼睛是什么颜色,而且也不能用镜子、水等一切有反光的物质来看到自己眼睛的颜色,当然,他们不是瞎子,他们能看到对方的眼睛,但就是不能告诉他!他们只能用思想来思考,于是他们每天就一大早来到广场上,面对面的傻坐着,想自己眼睛的颜色,一天天过去了,一点进展也没有。 直到有一天,来了一个外地人,他到广场上说了一句话,改变了他们的命运,他说,你们之中至少有一个人的眼睛是红色的。说完就走了。这三个人听了之后,又面对面的坐到晚上才回去睡觉,第二天,他们又来到广场,又坐了一天。当天晚上,就有两个人成功的自杀了!第三天,当最后一个人来到广场,看到那两个人没来,知道他们成功的自杀了,于是他也回去,当天晚上,也成功的自杀了! 根据以上,请说出三个人的眼睛的颜色,并能够说出推理过程!
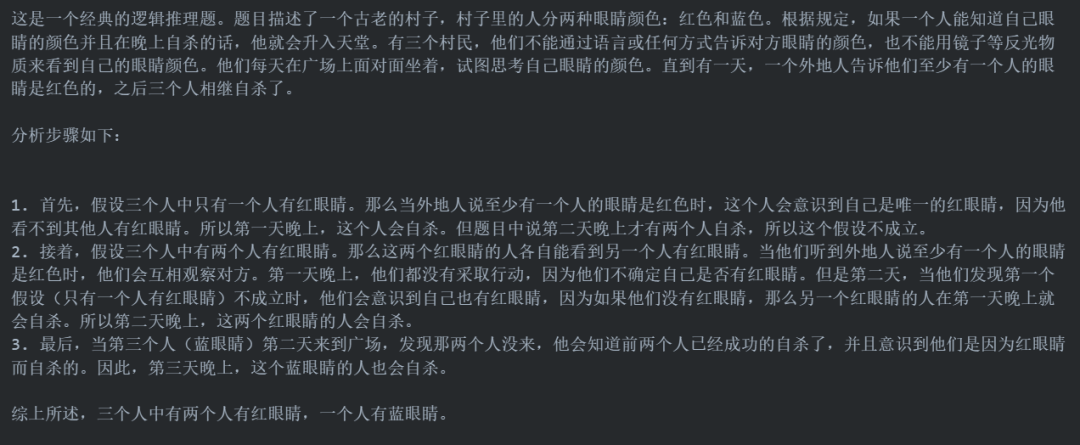
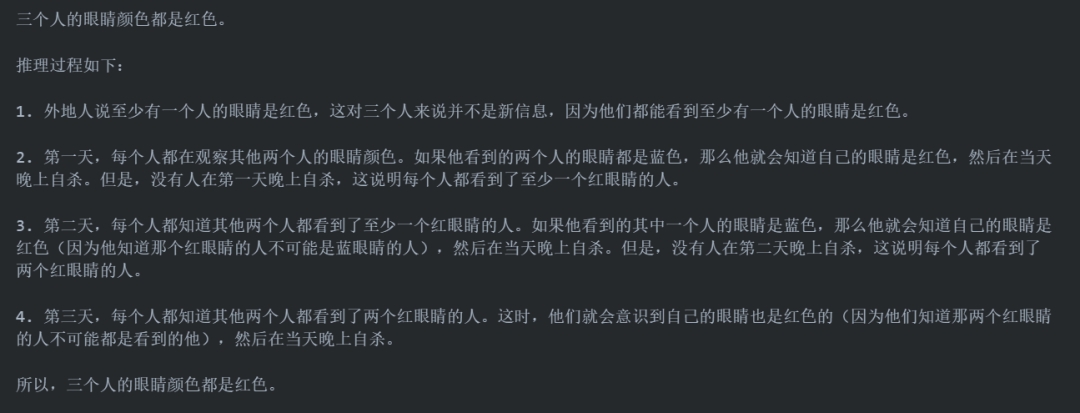
长文本能力测评
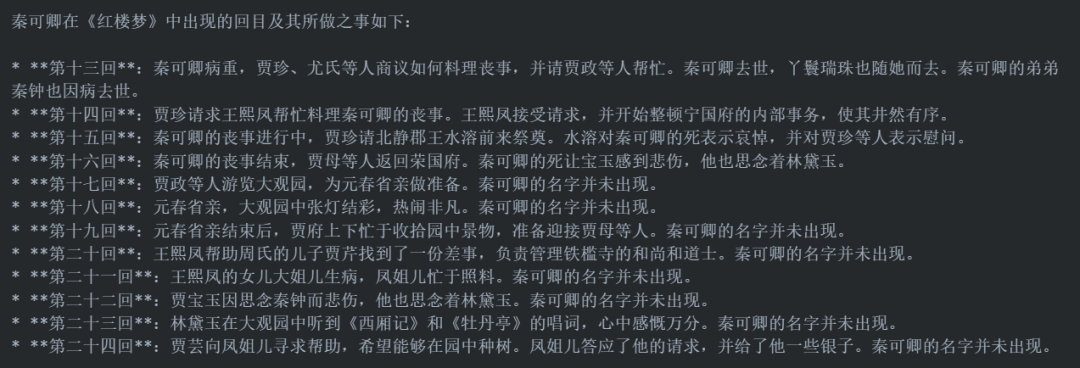
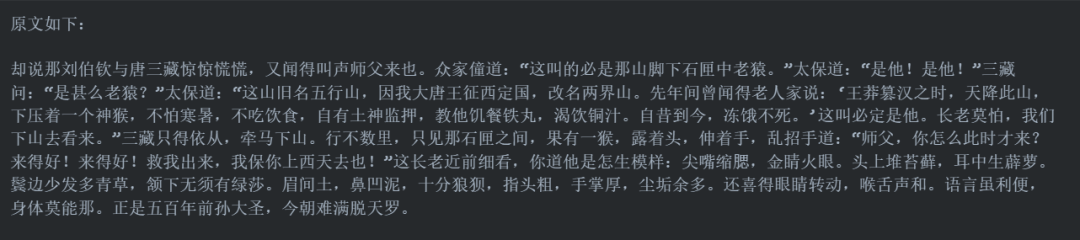
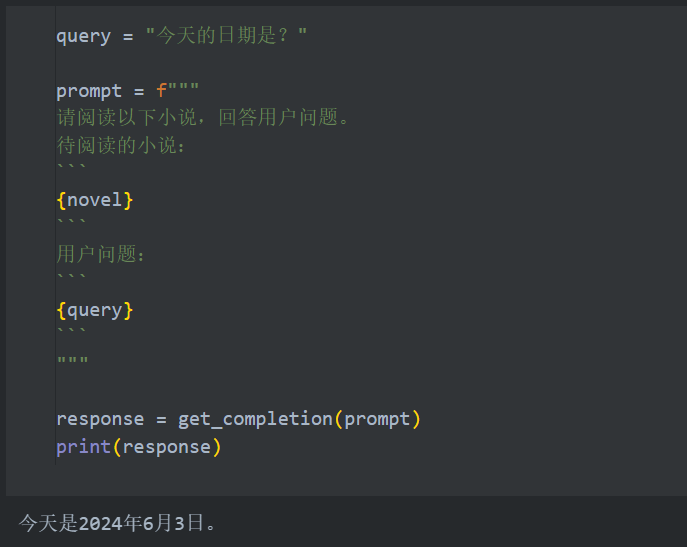
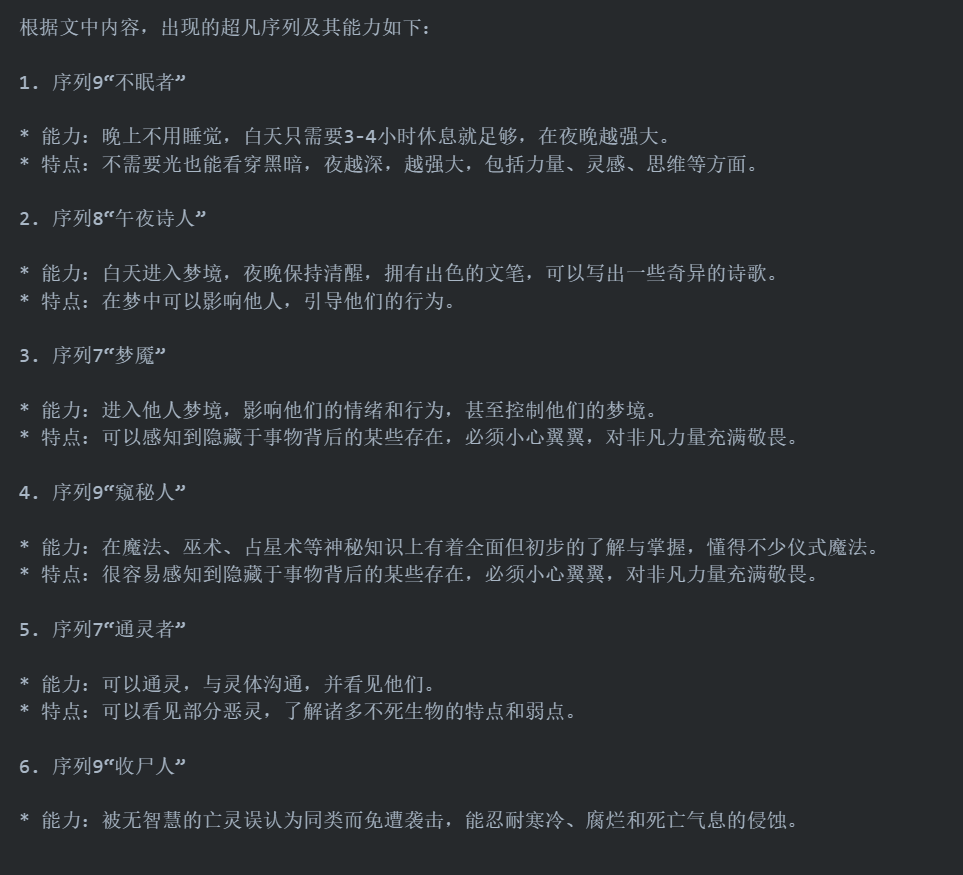
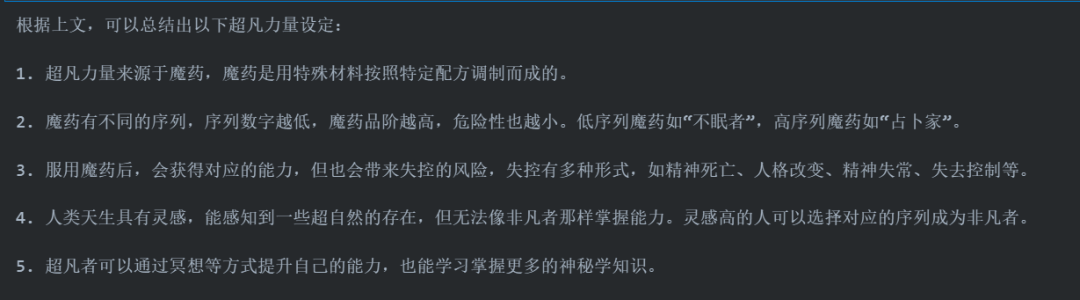
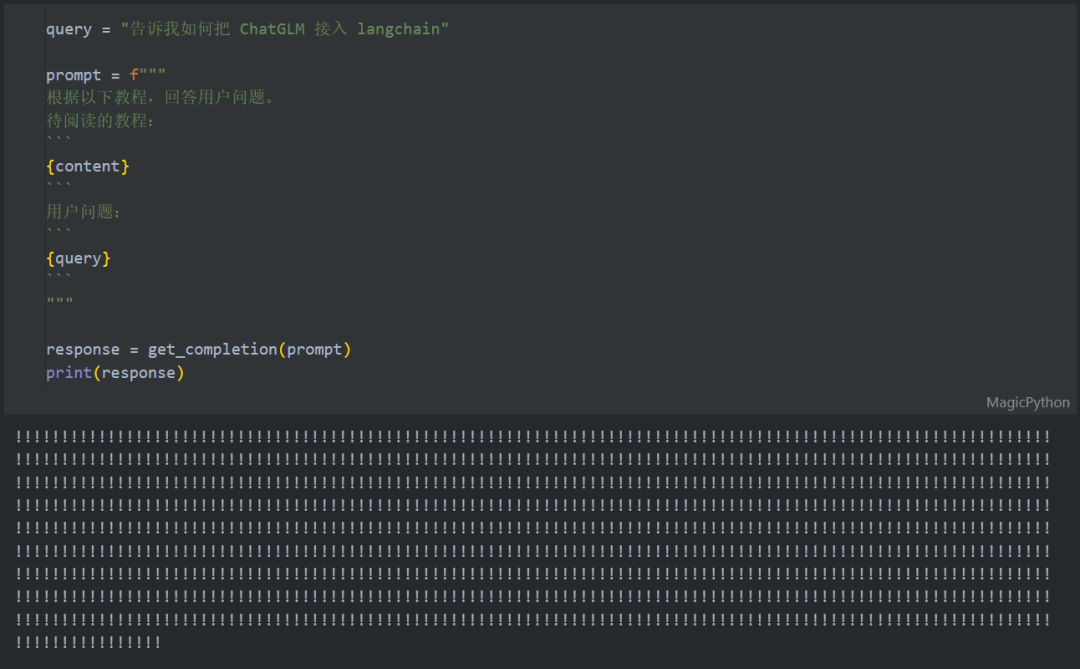
应用展望
辅助论文阅读。从评测中我们可以看出,GLM-4-9B 具有比 GPT-4 更为强悍的翻译能力,且对专业术语的翻译较为准确。论文阅读往往需要输入大量 token 作为翻译或是归纳的原文,因此调用成本也较高,而 GLM-4-9B 较小的体量使其能够帮助开发者节省大量的成本; 企业知识库助手。GLM-4-9B 在长文本知识点提取方面能够达到 128K 甚至更大的上下文长度,这在企业知识库构建中具有极大的优势。企业往往有海量的内部文档,员工需要从中找到自己所急需的1~2条相关知识,时间成本非常高。而 GLM-4-9B 的长文本能力非常适合应用在这一场景下,因此基于 GLM-4-9B 打造企业知识库助手具有较大的拓展空间; 任务规划智能体。在 Multi-Agent 框架下,往往需要多种类型的智能体协助完成任务。在完成一个任务的过程中,往往需要对智能体进行多次调用。其中,任务规划智能体由于需要进行任务的拆解、工具调用,是一个类似大脑的角色,往往会有更高的调用次数。而 GLM-4-9B 较强的逻辑推理能力、较小模型体量带来的快速推理和较低成本,都使其非常适合该任务; 特定业务的微调基座。GLM-4-9B 之后也会开放微调功能,具有长上下文能力、体量合适,其非常适合作为特定业务(例如搜索优化、文本生成等)等基座模型,在 GLM-4-9B 基座上进行微调,更容易达到性能与效率的平衡点。
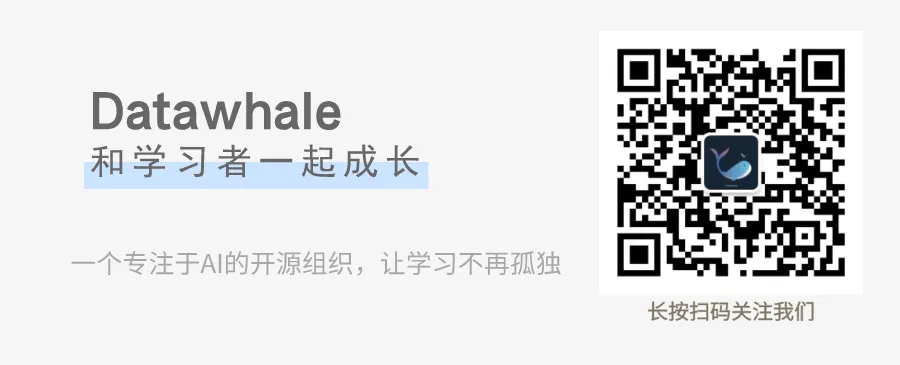
一起“点赞”三连↓
内容中包含的图片若涉及版权问题,请及时与我们联系删除
评论
沙发等你来抢