直播|CoRL X-Embodiment最佳论文奖,清华团队揭示具身智能Data Scaling Laws
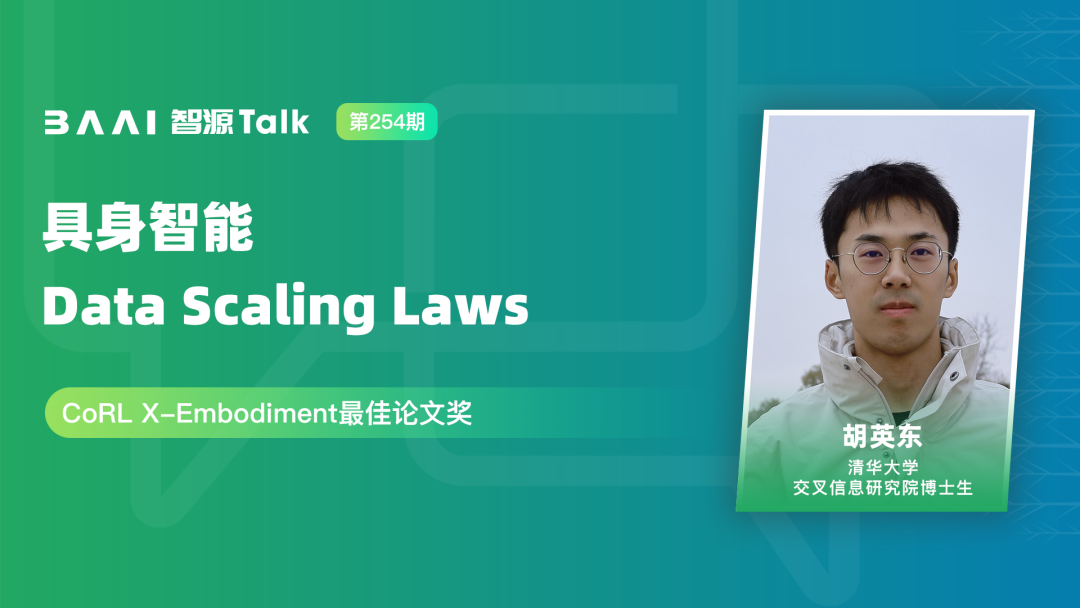
报告主题:CoRL X-Embodiment最佳论文奖,清华团队揭示具身智能Data Scaling Laws
报告日期:11月21日(周四)14:30-15:30
报告要点:
In this talk, we explore whether data scaling laws exist in robotics, particularly in robotic manipulation, and whether appropriate data scaling can yield single-task robot policies that can be deployed zero-shot for any object within the same category in any environment. To this end, we conduct a comprehensive empirical study on data scaling in imitation learning. By collecting data across numerous environments and objects, we study how a policy’s generalization performance changes with the number of training environments, objects, and demonstrations. Throughout our research, we collect over 40,000 demonstrations and execute more than 15,000 real-world robot rollouts under a rigorous evaluation protocol. Our findings reveal several intriguing results: the generalization performance of the policy follows a roughly power-law relationship with the number of environments and objects. The diversity of environments and objects is far more important than the absolute number of demonstrations; once the number of demonstrations per environment or object reaches a certain threshold, additional demonstrations have minimal effect. Based on these insights, we propose an efficient data collection strategy. With four data collectors working for one afternoon, we collect sufficient data to enable the policies for two tasks to achieve approximately 90% success rates in novel environments with unseen objects.报告嘉宾:
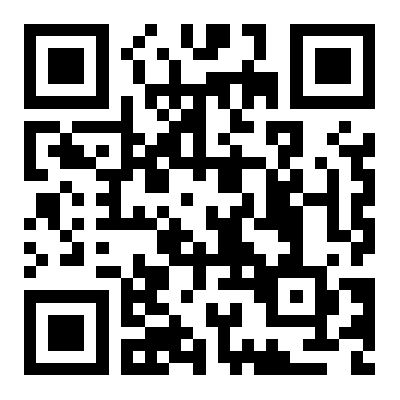
扫码报名
更多热门报告
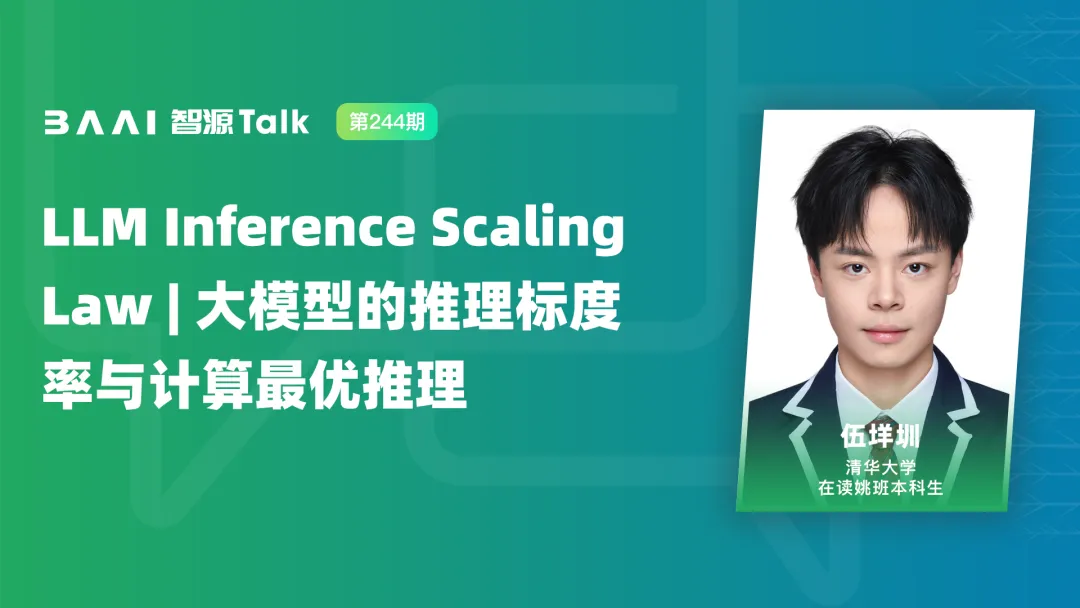
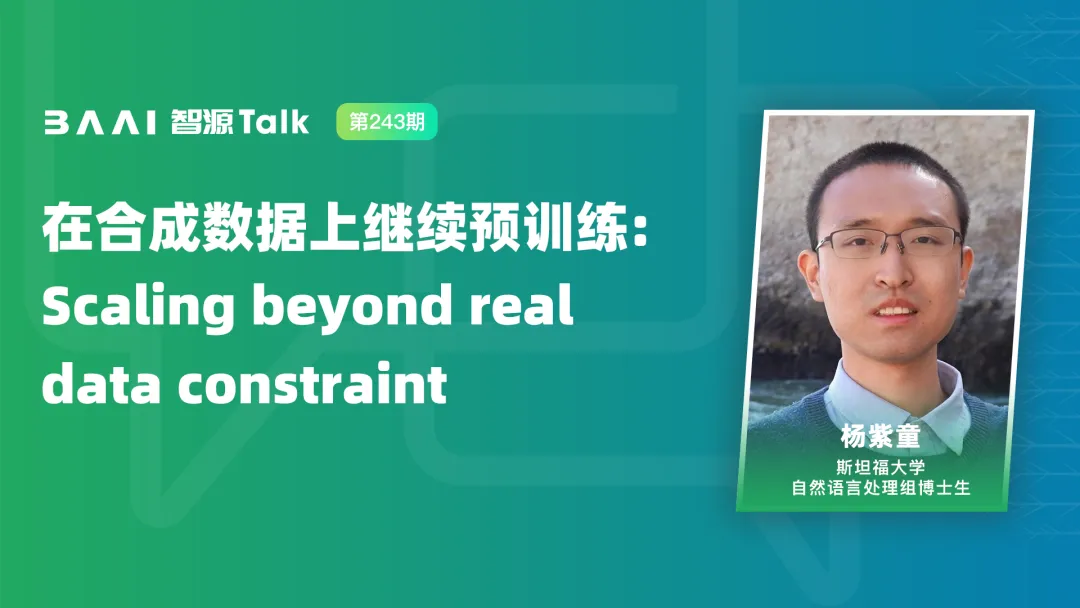
内容中包含的图片若涉及版权问题,请及时与我们联系删除
评论
沙发等你来抢