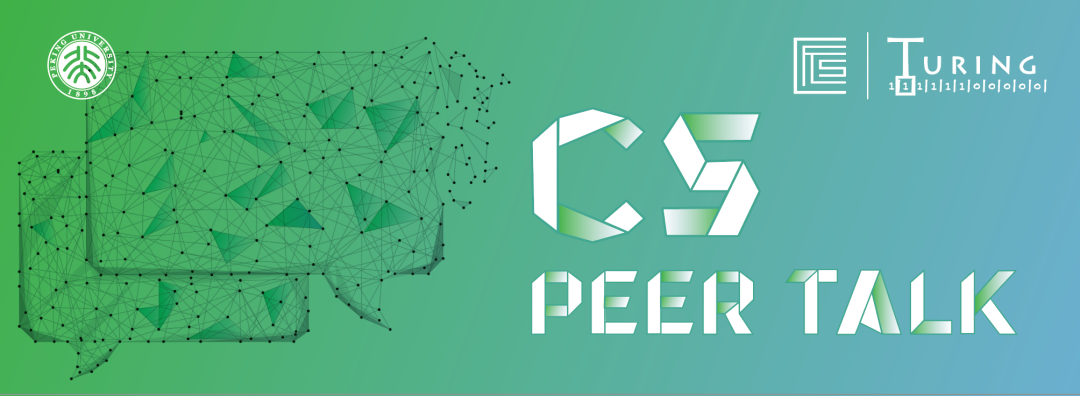
报告人:Weiqiang Zheng, Yale University
时间:5月28日(星期三)3:00pm
地点:静园五院204
Host:方嘉聪 图灵班2022级
Title
Efficient Online Learning and Equilibrium Computation in Non-Concave Games
Abstract
Many modern applications and outstanding challenges in machine learning can be modeled as games between multiple self-interested agents with high-dimension and non-concave utilities. Examples include systems with explicit strategic behaviors like multi-agent reinforcement learning and auto-bidding in advertising auctions and ML applications that can be implicitly modeled as games like training generative models and robust optimization. This new multi-agent learning paradigm emerges as non-concave games, introduce significant game-theoretic and optimization challenges: (1) Nash equilibria may not exist; (2) local Nash equilibria exist but are computationally intractable; (3) mixed Nash, (coarse) correlated equilibria generally have infinite support and are intractable. To sidestep these challenges, we consider the classical solution concept of
When
is finite, we give an efficient randomized online learning algorithm to minimize -regret, thus giving efficient uncoupled learning dynamics for approximate -equilibria. When
is infinite, we give a hardness result even when contains only local deviations. This result also implies the NP-hardness of computing an approximate local minimizer of a quadratic function over a polytope. We also uncover a surprising and previously unrecognized property of the widely used algorithm online gradient descent, demonstrating its ability to minimize a new class of regret—proximal regret—which generalizes external regret as a special case.
This talk is based on a joint work with Yang Cai, Costis Daskalakis, Haipeng Luo, and Chen-Yu Wei.
Biography
Weiqiang Zheng is a fourth-year computer science PhD student at Yale University, advised by Prof. Yang Cai. He received his bachelor's degree from Turing Class, Peking University. His has a broad interest in algorithmic game theory, online learning, and optimization. His recent research focuses on developing fast algorithms for minimax optimization, reinforcement learning, and learning in games, as well as their applications for LLM alignment. He has been recognized as a 2025 KAUST rising star in AI.
about CS Peer Talk
作为活动的发起人,我们来自北京大学图灵班科研活动委员会,主要由图灵班各年级同学组成。我们希望搭建一个CS同学交流的平台,促进同学间的交流合作,帮助同学练习展示,同时增进友谊。
目前在计划中的系列包括但不限于:
教程系列:学生讲者为主,介绍自己的研究领域
研究系列:学生讲者为主,介绍自己的研究成果
客座系列:邀请老师做主题报告
除非报告人特别要求,报告默认是非公开的,希望营造一个自由放松但又互相激励的交流氛围。
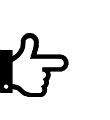
如果你愿意和大家分享你的学术成果、经历经验,总结回顾、触发新思,欢迎报名自荐。
主讲人报名:发邮件至 cs_research_tc@163.com,并抄送 cfcs@pku.edu.cn,写明想讲的题目、内容及时间。
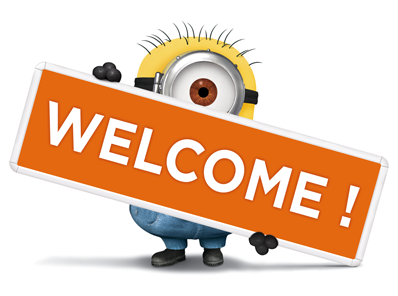
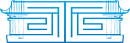
北京大学图灵班科研活动委员会

— 版权声明 —
本微信公众号所有内容,由北京大学前沿计算研究中心微信自身创作、收集的文字、图片和音视频资料,版权属北京大学前沿计算研究中心微信所有;从公开渠道收集、整理及授权转载的文字、图片和音视频资料,版权属原作者。本公众号内容原作者如不愿意在本号刊登内容,请及时通知本号,予以删除。
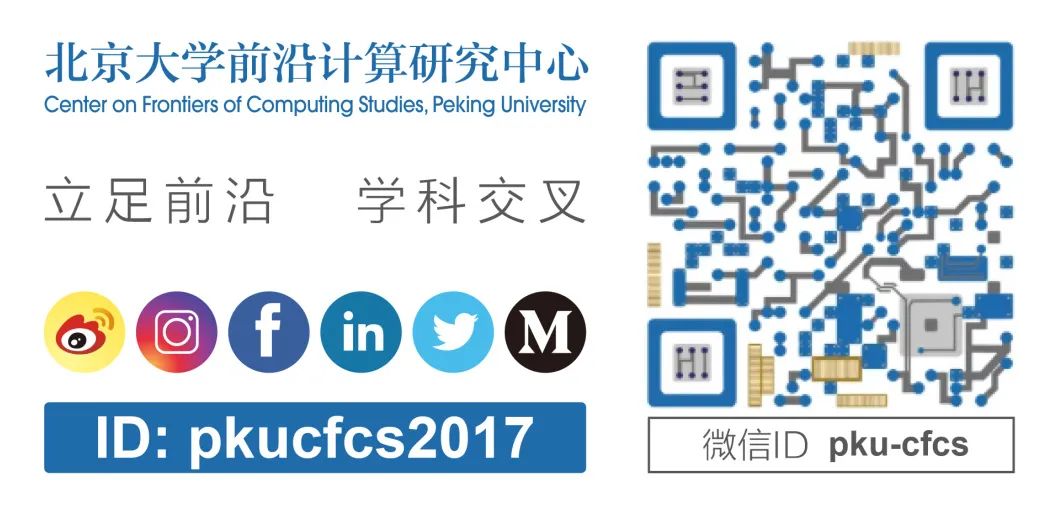
内容中包含的图片若涉及版权问题,请及时与我们联系删除
评论
沙发等你来抢